Urban Day-to-Day Travel and Its Development in an Information Environment: A Review
[ad_1]
1. Introduction
Scholars have already conducted extensive theoretical research on micro travel decision-making of urban day-to-day travelers and macro road traffic flow changes, making necessary contributions to the modeling of urban day-to-day travel. However, there are few studies that systematically analyze the methodologies that have been proposed by scholars and organize the development of these methodologies. In addition, the advent of the information age is bringing and will continue to bring new impacts on the day-to-day travel decisions of travelers by providing various types of travel reference information, which will become increasingly important for the development and change in urban day-to-day travel systems in the future. In this context, conducting a phased review of the theoretical research on urban day-to-day travel and the impact of the information environment on it is of great importance for grasping the effective analytical methodology of day-to-day travel patterns in the information age.
2. Information Environment for Urban Day-to-Day Travel
2.1. The Connotation of Information Environment
Information factors have existed since the birth of urban day-to-day travel systems. The travel experience of travelers in route choice is just a kind of information that will affect their micro decision-making regarding their next travel decision-making. After travel decisions have been made, new traffic information will emerge in urban day-to-day travel systems, and travelers as individuals will also acquire new travel experience information. However, these pieces of information are relatively isolated. The emergence of the information environment is derived from the continuous development of information and communication technology (ICT). With the help of ICT, urban day-to-day travelers can access traffic information through various types of public information dissemination channels.
2.2. Developing Tendency of Urban Day-to-Day Travel System in Information Environment
3. Development of Micro Travel Decision-Making in Information Environment
3.1. Traditional Models
3.1.1. Deterministic Models
3.1.2. Stochastic Models
In the study of micro travel decision-making, many scholars also believe that the decisions made by each individual in urban day-to-day travel systems under the impact of multiple complex factors have strong randomness. Therefore, a large number of stochastic models have been proposed to describe micro travel decision-making in day-to-day travel, including the random utility model (RUM), the stochastic learning model, the Markov random state transition theory-based model, and the reinforcement learning (RL)-based model.
3.2. Models with Information Factors Considered
3.3. The Impact of Information Environment on Micro Travel Decisions
3.3.1. Positive Impact of Traffic Information on Micro Travel Decisions
3.3.2. Negative Impact of Traffic Information on Micro Travel Decisions
As mentioned at the beginning of this section, the introduction of traffic information into day-to-day travel is not totally positive, according to some practical tests. The publication of certain traffic information often has side effects on travel decisions and may not even provide significant and effective assistance for the overall governance of urban day-to-day travel systems, leading to negative attitudes among both travelers and urban traffic-governing officials towards the acceptance of traffic information.
3.3.3. Dialectical Discussion of Traffic Information on Micro Travel Decisions
4. Development of Macro Traffic Flow Changes in Information Environment
Nevertheless, despite all the difficulties mentioned above, scholars have still made many efforts to explain macro road traffic flow changes in urban day-to-day travel. They have formed many representative achievements in analyzing the impact of micro travel decisions on macro phenomena, the characteristics of macro traffic flow, and even the effective explanation methods of macro traffic flow in an information environment.
4.1. Traditional Explanations
For the effective explanation of macro traffic flow under the impact of micro travel decisions in urban day-to-day travel systems, traditional explanations have mainly focused on macro traffic flow state, which includes not only the qualitative phenomena but also the state of micro travelers’ travel patterns and their appearance in various road segments in the macro road network at different travel times.
4.1.1. Qualitative Macro Phenomena Explanations
4.1.2. Quantitative Macro Traffic Flow Explanations
4.2. Explanations with Information Factors Considered
4.3. The Impact of Information Environment on Macro Traffic Flow
The macro urban transportation system, represented by the urban road traffic network, is not a direct recipient of traffic information in day-to-day operations but only passively changes in the form of traffic flow through aggregation effects after micro travelers carry out their travel activities. Therefore, there is not much research on the impact of traffic information on macro road traffic flow changes compared to the research on the impact of traffic information on micro travel decision-making.
5. Development of Information Environment Understanding
With the constant development of various intelligent systems and terminals that create urban day-to-day travel systems, scholars also tend to have a deeper understanding of the information environment. The types of traffic information provided to urban day-to-day travelers in an information environment can be further divided into global and local traffic information according to the range of travelers obtained. Global traffic information, as it is called, can be obtained through various public information dissemination channels, such as road flow status, traffic control strategies, etc., whereas local traffic information is released through certain channels and only available to some travelers, such as sudden congestion and control information, on certain parts of the urban road network. The impacts of different types of traffic information on micro travel decision-making are different, and, in turn, their impacts on the distribution of macro traffic flow also vary.
5.1. The Impact of Global Traffic Information on Day-to-Day Travelers
The most common type of information that urban day-to-day travelers can access in an information environment is travel-related information that can be obtained through various public information dissemination channels. In this section, it is defined as global traffic information. In the old era, when smartphones and intelligent terminals were not yet fully popularized, the scope of global traffic information was relatively narrow. At that time, specific urban traffic governance strategies known to day-to-day travelers, such as road congestion pricing, were types of important global information that affected their travel strategies.
5.2. The Impact of Local Traffic Information on Day-to-Day Travelers
The complexity of urban day-to-day travel systems in an information environment determines that all participants in the system may not be able to access the same travel reference information at all times. This phenomenon has a local impact on urban day-to-day travel. It may be caused by local failures of the urban travel system (such as sudden road accidents, local operational failures of the public transportation system, etc.) or by the formation of travel groups among several travelers and collective travel decisions. Scholars have noticed such issues and have already conducted a series of related studies.
6. Open Issues
6.1. Using Increasingly Diverse Information Technology Methods for Day-to-Day Travel
The continuous penetration of information technology has made it increasingly difficult to understand the development and changes taking place in urban day-to-day travel systems. In this context, effective governance of urban travel systems should take the initiative to embrace information. The penetration of information technology into urban day-to-day travel systems should be seen as a “double-edged sword”. While information technology is increasing the complexity of the system, it also provides new opportunities for using new data acquisition and analysis methodologies in the information age to solve difficult problems in urban traffic governance.
There is reason to believe that with the increase in the dimensions of day-to-day travel data resources available in the information age and the advancement of methodologies for processing massive amounts of data, scholars will also propose more competitive methodologies to explore those issues related to the laws of urban day-to-day travel systems that cannot be solved by traditional travel surveys.
6.2. Deep Integration of Micro Travel Decision-Making and Macro Traffic Analysis
From the relevant research on modeling urban day-to-day travel and the impact of the information environment on it in the previous sections, it can be found that there are two clear main lines in the relevant research. The first one is to discuss the travel decision-making of micro travelers, and the second one is to discuss the macro aggregation effects of day-to-day travel. However, as urban day-to-day travel systems can be recognized as complex systems, their macro and micro analyses should not be completely separated. Scholars have also discovered this issue and have attempted to pursue a deep integration of micro travel decision-making and macro traffic analysis.
From the previous discussion, it can be seen that although macro and micro integrated modeling of day-to-day travel systems has been implemented, scholars have only made some attempts, but it is still not systematic. To be specific, the mainstream of urban day-to-day travel systems is still in three main topics: (1) micro travel decisions and their influencing factors, (2) macro traffic flow change analysis, and (3) the analysis of the advantages and disadvantages of an information environment for travel systems. The analysis of urban day-to-day travel systems in an information environment urgently requires the support of emerging theories, breaking the current situation where research studies in the three main topics are all on their own. And it can be expected that the deep integration of micro travel decision-making and macro traffic analysis would be the focus of urban day-to-day travel-related research.
6.3. Developing Novel Theories to Explain Urban Day-to-Day Travel Systems
7. Conclusions
Urban day-to-day travel systems are complex systems with almost all of the complex system characteristics. After introducing traffic information, with the diversification of information exchange channels between travelers and macro road networks, the complexity of urban day-to-day travel systems is further increased. Understanding the complex urban day-to-day travel system is of great significance for the sustainable development of cities in terms of traffic governance. This paper first reviews the traditional micro decision-making of urban day-to-day travel and the macro road traffic flow analysis as the aggregation effect of micro travel decisions, clarifying some representative methodologies commonly used in these specific research problems. Subsequently, this paper clarifies that in the information era, the ways of obtaining traffic information have become increasingly diverse, and the introduction of traffic information can bring profound changes to urban day-to-day travel systems. After commenting on this, a dialectical discussion has been conducted on the potential impact of traffic information on micro travel decisions and macro traffic flow changes in urban day-to-day travel systems. Finally, some open issues regarding the future theoretical development direction of urban day-to-day travel systems in an information environment have been given, hoping to arouse higher research interest among scholars in this field.
Due to the lack of systematic discussion in previous review papers on the methodologies of explaining urban day-to-day travel systems, let alone systematic discussion of urban day-to-day travel systems in an information environment, the main contribution of this paper is just a systematic review of the traditional theoretical development of urban day-to-day travel systems, as well as the innovative theoretical development of urban day-to-day travel systems in an information environment after introducing traffic information. In addition, this paper has also pointed out several challenging open issues for the future theoretical development of urban day-to-day travel systems. Of course, considering the ubiquitous and rapid development of ICT in the current lives of urban residents, the relevant research reviewed in this paper only extends to the widespread use of smartphones and smart terminals among travelers and the information environment created by the coexistence of traditional travel information acquisition media, various ITS-related applications, and online social media. With the further development of ICT, there will be new and unpredictable changes in the travel decisions of urban day-to-day travelers and even the future development of urban day-to-day travel systems.
In addition, in the discussion of this paper, relevant, innovative work being carried out on urban day-to-day travel systems in an information environment has also been pointed out. Firstly, scholars have found that instead of passively viewing traffic information as a factor that increases the complexity of urban day-to-day travel systems, it is better to actively view it as a tool for fitting higher fidelity OD pairs and judging travel modes between them. Secondly, scholars have found that discussing micro-level travel decisions and macro-level traffic flow changes separately is increasingly insufficient to objectively explain the development of and changes in urban day-to-day travel in an information environment; deep integration of the research on both macro and micro levels is underway. Moreover, scholars are striving to explore new theories that can provide more effective explanations for urban day-to-day travel systems in an information environment; emerging theories such as complex system dynamics and quantum mechanics are the most representative and promising ones.
Through the review of this paper, some beneficial insights can be given to the management and governance departments of contemporary urban travel systems: firstly, the governance of urban travel systems in an information environment should be one of the core tasks of long-term urban sustainable development; secondly, if information technology can be fully utilized to solve the source problem of traffic survey data, the “knowledge” contained in day-to-day travel data can be fully explored through various emerging algorithms in the information age; finally, for day-to-day travel systems in an information environment, from the classification of personality differences in the micro travel decision-making process of individual travelers to the long-term and short-term analysis of macro road traffic flow-changing processes under the influence of micro travel decisions, and even to the establishment of a macro and micro integrated theoretical analysis system, these are all needed to break away from the traditional single discipline of transportation engineering in seeking proper theoretical explanations and establishing new theoretical systems.
Author Contributions
Conceptualization, W.N.; methodology, W.N. and D.L.; investigation, W.N., Z.Y., L.L., Y.F. and Y.G.; writing—original draft preparation, W.N. and Z.Y.; writing—review and editing, D.L.; supervision, W.N.; funding acquisition, W.N., Z.Y. and D.L. All authors have read and agreed to the published version of the manuscript.
Funding
This work was supported in part by the General Scientific Research Fund of Zhejiang Provincial Education Department under Grant No. Y202351125, in part by the Huzhou Natural Science Fund Project under Grant No. 2023YZ35, and in part by the Initial Scientific Research Fund of Talent Introduction in Huzhou College under Grant No. RK59004, No. RK59006 and No. RK64001.
Institutional Review Board Statement
Not applicable.
Informed Consent Statement
Not applicable.
Data Availability Statement
Not applicable.
Conflicts of Interest
The authors declare no conflicts of interest.
References
- Chapin, F.S. Human Activity Patterns in the City: Things People Do in Time and in Space; Wiley & Sons: New York, NY, USA, 1974; pp. 178–180. [Google Scholar]
- Juan, Z.; Xianyu, J. Research on activity-travel decision behavior with effect of traffic information. China J. Highw. Transp. 2008, 21, 88–93. [Google Scholar]
- Li, D.; Miwa, T.; Xu, C.; Li, Z. Non-linear fixed and multi-level random effects of origin-destination specific attributes on route choice behaviour. IET Intell. Transp. Syst. 2019, 13, 654–660. [Google Scholar] [CrossRef]
- Wang, Z.; Lin, W.H.; Xu, W. A data driven approach to assessing the reliability of using taxicab as probes for real-time route selections. J. Intell. Transp. Syst. 2021, 25, 331–342. [Google Scholar] [CrossRef]
- Mahmassani, H.S.; Liu, Y.H. Dynamics of commuting decision behaviour under advanced traveller information systems. Transp. Res. Part C Emerg. Technol. 1999, 7, 91–107. [Google Scholar] [CrossRef]
- Zandbergen, P.A. Accuracy of iPhone locations: A comparison of assisted GPS, WiFi and cellular positioning. Trans. GIS 2009, 13, 5–25. [Google Scholar] [CrossRef]
- Simon, R.; Frohlich, P.; Grechenig, T. GeoPointing: Evaluating the performance of orientation-aware location-based interaction under real-world conditions. J. Locat. Based Serv. 2008, 2, 24–40. [Google Scholar] [CrossRef]
- Schmid, F. Knowledge-based wayfinding maps for small display cartography. J. Locat. Based Serv. 2008, 2, 57–83. [Google Scholar] [CrossRef]
- Ratti, C.; Frenchman, D.; Pulselli, R.M.; Williams, S. Mobile landscapes: Using location data from cell phones for urban analysis. Environ. Plan. B Plan. Des. 2006, 33, 727–748. [Google Scholar] [CrossRef]
- Arminen, I. Social functions of location in mobile telephony. Pers. Ubiquitous Comput. 2006, 10, 319–323. [Google Scholar] [CrossRef]
- May, A.; Bayer, S.H.; Ross, T. A survey of “young social” and “professional” users of location-based services in the UK. J. Locat. Based Serv. 2007, 1, 112–132. [Google Scholar] [CrossRef]
- Klippel, A.; Tappe, H.; Kulik, L.; Lee, P.U. Wayfinding choremes—A language for modeling conceptual route knowledge. J. Vis. Lang. Comput. 2005, 16, 311–329. [Google Scholar] [CrossRef]
- Bhat, C.R.; Sivakumar, A.; Axhausen, K.W. An analysis of the impact of information and communication technologies on non-maintenance shopping activities. Transp. Res. Part B Methodol. 2003, 37, 857–881. [Google Scholar] [CrossRef]
- Kwan, M.P. Mobile communications, social networks, and urban travel: Hypertext as a new metaphor for conceptualizing spatial interaction. Prof. Geogr. 2007, 59, 434–446. [Google Scholar] [CrossRef]
- Line, T.; Jain, J.; Lyons, G. The role of ICTs in everyday mobile lives. J. Transp. Geogr. 2010, 19, 1490–1499. [Google Scholar] [CrossRef]
- Watling, D.; Hazelton, M.L. The dynamics and equilibria of day-to-day assignment models. Netw. Spat. Econ. 2003, 3, 349–370. [Google Scholar] [CrossRef]
- Watling, D. Urban traffic network models and dynamic driver information systems. Transp. Rev. 1994, 14, 219–246. [Google Scholar] [CrossRef]
- Bogers, E.A.I.; Viti, F.; Hoogendoorn, S.P. Joint modeling of advanced travel information service, habit and learning impacts on route choice by laboratory simulator experiments. Transp. Res. Rec. 2005, 1926, 189–197. [Google Scholar] [CrossRef]
- Nakayama, S.; Kitamura, R. Route choice model with inductive learning. Transp. Res. Rec. 2000, 1725, 63–70. [Google Scholar] [CrossRef]
- Nakayama, S.; Kitamura, R.; Fujii, S. Drivers’ route choice rules and network behavior: Do drivers become rational and homogeneous through learning? Transp. Res. Rec. 2001, 1752, 62–68. [Google Scholar] [CrossRef]
- Nakayama, S.; Kitamura, R.; Fujii, S. Drivers’ learning and network behavior dynamic analysis of the driver-network system as a complex system. Transp. Res. Rec. 1999, 1676, 30–36. [Google Scholar] [CrossRef]
- Watling, D. Stability of the stochastic equilibrium assignment problem: A dynamical systems approach. Transp. Res. Part B Methodol. 1999, 33, 281–312. [Google Scholar] [CrossRef]
- Ye, H.; Xiao, F.; Yang, H. Exploration of day-to-day route choice models by a virtual experiment. Transp. Res. Part C Emerg. Technol. 2018, 94, 220–235. [Google Scholar] [CrossRef]
- Yang, F.; Zhang, D. Day-to-day stationary link flow pattern. Transp. Res. Part B Methodol. 2009, 43, 119–126. [Google Scholar] [CrossRef]
- Smith, M.J. The existence and calculation of traffic equilibria. Transp. Res. Part B Methodol. 1983, 17, 291–301. [Google Scholar] [CrossRef]
- Smith, M.J. The stability of a dynamic model of traffic assignment—An application of a method of Lyapunov. Transp. Sci. 1984, 18, 245–252. [Google Scholar] [CrossRef]
- Smith, M.J.; Wisten, M.B. A continuous day-to-day traffic assignment model and the existence of a continuous dynamic user equilibrium. Ann. Oper. Res. 1995, 60, 59–79. [Google Scholar] [CrossRef]
- Huang, H.J.; Lam, W.H.K. Modeling and solving the dynamic user equilibrium route and departure time choice problem in network with queues. Transp. Res. Part B Methodol. 2002, 36, 253–273. [Google Scholar] [CrossRef]
- Peeta, S.; Yang, T.-H. Stability issues for dynamic traffic assignment. IFAC Proc. Vol. 2003, 33, 483–487. [Google Scholar] [CrossRef]
- Mounce, R. Convergence in a continuous dynamic queueing model for traffic networks. Transp. Res. Part B Methodol. 2006, 40, 779–791. [Google Scholar] [CrossRef]
- Mounce, R.; Carey, M. Route swapping in dynamic traffic networks. Transp. Res. Part B Methodol. 2011, 45, 102–111. [Google Scholar] [CrossRef]
- Zhu, W.; Ma, S.; Tian, J.; Li, G. Nonlinear relative-proportion-based route adjustment process for day-to-day traffic dynamics: Modeling, equilibrium and stability analysis. Commun. Nonlinear Sci. Numer. Simul. 2016, 40, 129–137. [Google Scholar] [CrossRef]
- Alibabai, H.; Mahmassani, H.S. Foxes and sheep: Effect of predictive logic in day-to-day dynamics of route choice behavior. EURO J. Transp. Logist. 2016, 5, 53–67. [Google Scholar] [CrossRef]
- Friesz, T.L.; Bernstein, D.; Mehta, N.J.; Tobin, R.L.; Ganjalizadeh, S. Day-to-day dynamic network disequilibria and idealized traveler information systems. Oper. Res. 1994, 42, 1120–1136. [Google Scholar] [CrossRef]
- Jin, W.L. A dynamical system of the traffic assignment problem. Transp. Res. Part B Methodol. 2007, 41, 32–48. [Google Scholar] [CrossRef]
- Guo, R.Y.; Huang, H.J. Chaos and bifurcation in dynamical evolution process of traffic assignment with flow “mutation”. Chaos Solitons Fractals 2009, 41, 1150–1157. [Google Scholar] [CrossRef]
- Zhang, D.; Nagurney, A. On the local and global stability of a travel route choice adjustment process. Transp. Res. Part B Methodol. 1996, 30, 245–262. [Google Scholar] [CrossRef]
- Nagurney, A.; Zhang, D. Projected dynamical systems in the formulation, stability analysis, and computation of fixed-demand traffic network equilibria. Transp. Sci. 1997, 31, 147–158. [Google Scholar] [CrossRef]
- Zhong, R.; Li, X.; Liang, Q.; Chen, Z.; Pan, T. A projected Newton-like inertial dynamics for modeling day-to-day traffic evolution with elastic demand. Transp. A Transp. Sci. 2024, in press. [CrossRef]
- Bertsekas, D.P.; Gafni, E.M. Projection methods for variational inequalities with application to the traffic assignment problem. Math. Program. Study 1982, 17, 139–159. [Google Scholar]
- Davis, G.; Nihan, N. Large population approximations of a general stochastic traffic assignment model. Oper. Res. 1993, 41, 169–178. [Google Scholar] [CrossRef]
- Sandholm, W.H. Potential games with continuous player sets. J. Econ. Theory 2001, 97, 81–108. [Google Scholar] [CrossRef]
- Lotan, T. Effects of familiarity on route choice behavior in the presence of information. Transp. Res. Part C Emerg. Technol. 1997, 5, 225–243. [Google Scholar] [CrossRef]
- Ozbay, K.; Datta, A.; Kachroo, P. Application of stochastic learning automata for modeling departure time and route choice behavior. Transp. Res. Rec. 2002, 1807, 154–162. [Google Scholar] [CrossRef]
- Ozbay, K.; Kachroo, P.; Datta, A. Modeling route choice behavior with stochastic learning automata. Transp. Res. Rec. 2002, 1752, 38–46. [Google Scholar] [CrossRef]
- Helzelton, M.L. Day-to-day variation in Markovian traffic assignment models. Transp. Res. Part B Methodol. 2002, 36, 637–648. [Google Scholar] [CrossRef]
- Hazelton, M.; Watling, D. Computation of equilibrium distributions of markov traffic assignment models. Transp. Sci. 2004, 38, 331–342. [Google Scholar] [CrossRef]
- Wahba, M.; Shalaby, A. Large-scale application of MILATRAS: Case study of the Toronto transit network. Transportation 2011, 38, 889–908. [Google Scholar] [CrossRef]
- Wahba, M.; Shalaby, A. Learning-based framework for transit assignment modeling under information provision. Transportation 2014, 41, 397–417. [Google Scholar] [CrossRef]
- Zolfpour-Arokhlo, M.; Selamat, A.; Hashim, S.Z.M.; Afkhami, H. Modeling of route planning system based on Q value-based dynamic programming with multi-agent reinforcement learning algorithms. Eng. Appl. Artif. Intell. 2014, 29, 163–177. [Google Scholar] [CrossRef]
- Horowitz, J.L. The stability of stochastic equilibrium in a two-link transportation network. Transp. Res. Part B Methodol. 1984, 18, 13–28. [Google Scholar] [CrossRef]
- Cantarella, G.E.; Cascetta, E. Dynamic processes and equilibrium in transportation networks: Towards a unifying theory. Transp. Sci. 1995, 29, 305–329. [Google Scholar] [CrossRef]
- Jha, M.; Madanat, S.; Peeta, S. Perception updating and day-to-day travel choice dynamics in traffic networks with information provision. Transp. Res. Part C Emerg. Technol. 1998, 6, 189–212. [Google Scholar] [CrossRef]
- Hall, R.W. Travel outcome and performance: The effect of uncertainty on accessibility. Transp. Res. Part B Methodol. 1983, 17, 275–290. [Google Scholar] [CrossRef]
- Abdelaty, M.A.; Kitamura, R.; Jovanis, P.P. Investigating effect of travel time variability on route choice using repeated-measurement stated preference data. Transp. Res. Rec. 1995, 1493, 39–45. [Google Scholar]
- Huang, H.; Li, Z. A multiclass multicriteria Logit-based traffic equilibrium assignment model under ATIS. Eur. J. Oper. Res. 2007, 176, 1464–1477. [Google Scholar] [CrossRef]
- Li, Z.; Huang, H.; Lam, W.H.K.; Wong, S.C. A model for evaluation of transport policies in multimodal networks with road and parking capacity constraints. J. Math. Model. Algorithms 2007, 6, 239–257. [Google Scholar] [CrossRef]
- Guo, H. Study on Travelers’ Behavior Based on Their Physiology and Psychology in Urban Passenger Transportation. Ph.D. Thesis, Southwest Jiaotong University, Chengdu, China, 2007. [Google Scholar]
- Fan, W.; Chun, Z.; Jiang, G. Modeling of commuter’s day-to-day route choice behaviors based on reference-dependent approach. J. Traffic Transp. Eng. 2009, 9, 96–99. [Google Scholar] [CrossRef]
- Fan, W.; Li, Z. Modeling commuters’ day-to-day route choice dynamics under network uncertainty: A reference-dependent approach. Dyn. Contin. Discret. Impuls. Syst. Ser. B Appl. Algorithms 2009, 16, 151–165. [Google Scholar]
- Li, M.; Lu, J.; Sun, J.; Tu, Q. Day-to-day evolution of traffic flow with dynamic rerouting in degradable transport network. J. Adv. Transp. 2019, 2019, 1524178. [Google Scholar] [CrossRef]
- Khattak, A.J.; Koppelmen, F.S.; Schofer, J.L. Stated preferences for investigating commuters diversion propensity. Transportation 1993, 20, 107–127. [Google Scholar] [CrossRef]
- Cheng, Q.; Deng, W.; Raza, M.A. Analysis of the departure time choices of metro passengers during peak hours. IET Intell. Transp. Syst. 2020, 14, 866–872. [Google Scholar] [CrossRef]
- Rossetti, R.J.F.; Bordini, R.H.; Bazzan, A.L.C.; Bampi, S.; Liu, R.; Vliet, D.V. Using BDI agents to improve driver modeling in a commuter scenario. Transp. Res. Part C Emerg. Technol. 2002, 10, 373–398. [Google Scholar] [CrossRef]
- Chen, R.; Mahmassani, H.S. Travel time perception and learning mechanisms in traffic networks. Transp. Res. Rec. 2004, 1894, 209–221. [Google Scholar] [CrossRef]
- Lahkar, R.; Seymour, R.M. Reinforcement learning in population games. Games Econ. Behav. 2013, 80, 10–38. [Google Scholar] [CrossRef]
- Wei, F.; Ma, S.; Jia, N. A day-to-day route choice model based on reinforcement learning. Math. Probl. Eng. 2014, 2014, 646548. [Google Scholar] [CrossRef]
- Nai, W.; Yang, Z.; Lin, D.; Li, D.; Xing, Y. A Vehicle Path Planning Algorithm Based on Mixed Policy Gradient Actor-Critic Model with Random Escape Term and Filter Optimization. J. Math. 2022, 2022, 3679145. [Google Scholar] [CrossRef]
- Zhao, Z.; Liang, Y. A deep inverse reinforcement learning approach to route choice modeling with context-dependent rewards. Transp. Res. Part C Emerg. Technol. 2023, 149, 104079. [Google Scholar] [CrossRef]
- Cui, Z.; Long, Y. Perspectives on stability and mobility of transit passenger’s travel behaviour through smart card data. IET Intell. Transp. Syst. 2019, 13, 1761–1769. [Google Scholar] [CrossRef]
- Kim, K. Effects of weather and calendar events on mode-choice behaviors for public transportation. J. Transp. Eng. Part A Syst. 2020, 146, 04020056. [Google Scholar] [CrossRef]
- Huang, Y.; Xiao, X.; Wang, D.; Jiang, H.; Wu, D. Exploring individual travel patterns across private car trajectory data. IEEE Trans. Intell. Transp. Syst. 2020, 21, 5036–5050. [Google Scholar] [CrossRef]
- Hadjidimitriou, N.S.; Cantelmo, G.; Antoniou, C. Machine learning for activity pattern detection. J. Intell. Transp. Syst. 2023, 27, 834–848. [Google Scholar] [CrossRef]
- Montello, D.R.; Davis, R.C.; Johnson, M.; Chrastil, E.R. The symmetry and asymmetry of pedestrian route choice. J. Environ. Psychol. 2023, 87, 102004. [Google Scholar] [CrossRef]
- Qi, H.; Jia, N.; Qu, X.; He, Z. Investigating day-to-day route choices based on multi-scenario laboratory experiments, Part I: Route-dependent attraction and its modeling. Transp. Res. Part A Policy Pract. 2023, 167, 103553. [Google Scholar] [CrossRef]
- Shi, X.; Wang, W.; Li, W. Information effects in transportation network. J. Syst. Eng. 2001, 16, 167–171. [Google Scholar] [CrossRef]
- Shi, X.; Wang, W.; Yang, D. Study of the effect of information on the travelers’ behavior. China J. Highw. Transp. 2002, 15, 89–92. [Google Scholar] [CrossRef]
- Shi, X. Study on dynamic choice model for ATIS. J. Syst. Eng. 2002, 17, 271–276. [Google Scholar] [CrossRef]
- Ben-Akiva, M.; de Palama, A.; Kaysi, I. Dynamic network models and driver Information systems. Transp. Res. Part A Policy Pract. 1991, 25, 251–266. [Google Scholar] [CrossRef]
- Adler, J.L.; Mcnally, M.G. In-laboratory experiments to investigate driver behavior under advanced traveler information system. Transp. Res. Part C Emerg. Technol. 1994, 2, 149–164. [Google Scholar] [CrossRef]
- Madanat, S.M.; Yang, C.V.D.; Yen, Y.M. Analysis of stated route diversion intentions under advanced traveler information systems using latent variable modeling. Transp. Res. Rec. 1995, 1485, 10–17. [Google Scholar]
- Bazzan, A.L.C.; Klügl, F. Case studies on the braess paradox: Simulating route recommendation and learning in abstract and microscopic models. Transp. Res. Part C Emerg. Technol. 2005, 13, 299–319. [Google Scholar] [CrossRef]
- Lila, P.C.; Anjaneyulu, M.V.L.R. Networkwide impact of telework in urban areas: Case study of Bangalore, India. J. Transp. Eng. Part A Syst. 2017, 143, 05017004. [Google Scholar] [CrossRef]
- Emmerink, R.H.M.; Verhoef, P.; Rietveld, P. Information provision in road transport with elastic demand: A welfare economic approach. J. Transp. Econ. Policy 1996, 30, 117–136. [Google Scholar]
- Wang, J.; Rakha, H. Empirical study of effect of dynamic travel time information on driver route choice behavior. Sensors 2020, 20, 3257. [Google Scholar] [CrossRef] [PubMed]
- Ben-Elia, E.; Shiftan, Y. Which road do I take? A learning based model of route-choice behavior with real-time information. Transp. Res. Part A Policy Pract. 2010, 44, 249–264. [Google Scholar] [CrossRef]
- Tsirimpa, A. Modeling the impact of traffic information aquisition from mobile devices during the primary tour of the day. J. Intell. Transp. Syst. 2015, 19, 125–133. [Google Scholar] [CrossRef]
- Arnott, R.; de Palma, A.; Lindsey, R. Does providing information to driver reduce traffic congestion? Transp. Res. Part A Gen. 1991, 25, 309–318. [Google Scholar] [CrossRef]
- Mannering, F.L. Poisson analysis of commuter flexibility in changing routes and departure times. Transp. Res. Part B Methodol. 1989, 23, 53–60. [Google Scholar] [CrossRef]
- Fusco, G.; Bracci, A.; Caligiuri, T.; Colombaroni, C.; Isaenko, N. Experimental analyses and clustering of travel choice behaviours by floating car big data in large urban area. IET Intell. Transp. Syst. 2018, 12, 270–278. [Google Scholar] [CrossRef]
- Yang, H. Multiple equilibrium behaviors and advance traveler information systems with endogenous market penetration. Transp. Res. Part B Methodol. 1998, 32, 205–218. [Google Scholar] [CrossRef]
- Yang, H.; Meng, Q. Modeling user adoption of advanced traveler information systems: Dynamic evolution and stationary equilibrium. Transp. Res. Part A Policy Pract. 2001, 35, 895–912. [Google Scholar] [CrossRef]
- Yin, Y.; Yang, H. Simultaneous determination of the equilibrium market penetration and compliance rate of advanced traveler information systems. Transp. Res. Part A Policy Pract. 2003, 37, 165–181. [Google Scholar] [CrossRef]
- van Essen, M.A. One Route or the Other? Master’s Thesis, University of Twente, Enschede, The Nethelands, 2014. [Google Scholar]
- Wu, W.; Huang, H. A study of modeling travelers’ route choice behavior and information release strategy. In Proceedings of the International Conference on Intelligent Computation Technology and Automation (ICICTA), Changsha, China, 20–22 October 2008. [Google Scholar] [CrossRef]
- Fan, A.; Chen, X.; Yu, L.; Li, M. Investigating heterogeneity in travel behavior change when implementing soft transport interventions: A latent class choice model. IET Intell. Transp. Syst. 2023, 17, 1072–1086. [Google Scholar] [CrossRef]
- Dia, H. An agent-based approach to modeling driver route choice behaviour under the influence of real-time information. Transp. Res. Part C Emerg. Technol. 2002, 10, 331–349. [Google Scholar] [CrossRef]
- Yang, H.; Huang, H.J. Modeling user adoption of advance traveler information systems: A control theoretic approach for optimal endogenous growth. Transp. Res. Part C Emerg. Technol. 2004, 12, 193–207. [Google Scholar] [CrossRef]
- Huang, H.J.; Liu, T.L.; Yang, H. Modeling the evolutions of day-to-day route choice and year-to-year ATIS adoption with stochastic user equilibrium. J. Adv. Transp. 2008, 42, 111–127. [Google Scholar] [CrossRef]
- Han, X.; Sun, Q.; Xing, Y.; Gao, Z.-Y.; Zhang, H.M. Reducing strategic uncertainty in transportation networks by personalized routing advice: A route-choice laboratory experiment. Travel Behav. Soc. 2024, 34, 100701. [Google Scholar] [CrossRef]
- Wei, F.; Jia, N.; Ma, S. Day-to-day traffic dynamics considering social interaction: From individual route choice behavior to a network flow model. Transp. Res. Part B Methodol. 2016, 94, 335–354. [Google Scholar] [CrossRef]
- Zhang, C.; Liu, T.-L.; Huang, H.-J.; Chen, J. A cumulative prospect theory approach to commuters’ day-to-day route-choice modeling with friends’ travel information. Transp. Res. Part C Emerg. Technol. 2018, 86, 527–548. [Google Scholar] [CrossRef]
- Diop, E.B.; Zhao, S.; Tran, V.D. Modeling travelers’ acceptance of variable message signs: A hierarchical hybrid choice model. J. Transp. Eng. Part A Syst. 2020, 146, 04020134. [Google Scholar] [CrossRef]
- Meneguzzer, C. Contrarians do better: Testing participants’ response to information in a simulated day-to-day route choice experiment. Travel Behav. Soc. 2019, 15, 146–156. [Google Scholar] [CrossRef]
- Meneguzzer, C. Day-to-day dynamics in a simple traffic network with mixed direct and contrarian route choice behaviors. Phys. A Stat. Mech. Its Appl. 2022, 603, 127841. [Google Scholar] [CrossRef]
- Ayaz, S.B.; Tian, H.; Gao, S.; Fisher, D.L. Proactive route choice with real-time information: Learning and effects of network complexity and cognitive load. Transp. Res. Part C Emerg. Technol. 2023, 149, 104035. [Google Scholar] [CrossRef]
- Zhao, X.; Wan, C.; Sun, H.; Xie, D.; Gao, Z. Dynamic rerouting behavior and its impact on dynamic traffic patterns. IEEE Trans. Intell. Transp. Syst. 2017, 18, 2763–2779. [Google Scholar] [CrossRef]
- Ilkhani, I.; Yazdanpanah, M.; Dehghanbanadaki, A. Effects of personality traits and visual health on driver route-choice behavior in response to VMS traffic information. Transp. Lett. 2023, 15, 1202–1217. [Google Scholar] [CrossRef]
- Zhou, B.; Xu, M.; Meng, Q.; Huang, Z. A day-to-day route flow evolution process towards in the mixed equilibria. Transp. Res. Part C Emerg. Technol. 2017, 82, 210–228. [Google Scholar] [CrossRef]
- Liu, S.; Guo, L.; Easa, S.M.; Yan, H.; Wei, H.; Tang, Y. Experimental study of dat-to-day route choice behavior: Evaluating the effect of ATIS market penetration. J. Adv. Transp. 2020, 2020, 8393724. [Google Scholar] [CrossRef]
- Rahimi-Farahani, H.; Rassafi, A.A.; Mirbaha, B. Forced-node route guidance system: Incorporating both user equilibrium and system optimal benefits. IET Intell. Transp. Syst. 2019, 13, 1851–1859. [Google Scholar] [CrossRef]
- Long, X.; Mao, J.; Qiao, Z.; Li, P.; He, W. Reinforcement learning of route choice considering traveler’s preference. Transp. Lett. 2024, in press. [CrossRef]
- Han, X.; Yu, Y.; Gao, Z.-Y.; Zhang, H.M. The value of pre-trip information on departure time and route choice in the morning commute under stochastic traffic conditions. Transp. Res. Part B Methodol. 2021, 152, 205–226. [Google Scholar] [CrossRef]
- Yu, Z.; Gayah, V.V. Simulation analysis of urban network performance under link disruptions: Impacts of information provisions in different street configurations. J. Intell. Transp. Syst. 2023, 27, 471–487. [Google Scholar] [CrossRef]
- Daganzo, C.F.; Sheffi, Y. On stochastic models of traffic assignment. Transp. Sci. 1977, 11, 253–274. [Google Scholar] [CrossRef]
- Mahmassani, H.; Chang, G.-L.; Herman, R. Individual decisions and collective effects in a simulated traffic system. Transp. Sci. 1986, 20, 227–291. [Google Scholar] [CrossRef]
- Friesz, T.; Shah, S. An overview of nontraditional formulations of static and dynamic equilibrium network design. Transp. Res. Part B Methodol. 2001, 35, 5–21. [Google Scholar] [CrossRef]
- Adler, J.L.; Blue, V.J. Toward the design of intelligent traveler information systems. Transp. Res. Part C Emerg. Technol. 1998, 6, 157–172. [Google Scholar] [CrossRef]
- Hu, T.Y.; Mahmassani, H.S. Day-to-day evolution of network flows under real-time information and reactive signal control. Transp. Res. Part C Emerg. Technol. 1997, 5, 51–69. [Google Scholar] [CrossRef]
- Chang, S.E.; Nojima, N. Measuring post-disaster transportation system performance: The 1995 Kobe earthquake in comparative perspective. Transp. Res. Part A Policy Pract. 2001, 35, 475–494. [Google Scholar] [CrossRef]
- Hunt, J.; Brownlee, A.; Stefan, K. Responses to centre street bridge closure: Where the “disappearing” travelers went. Transp. Res. Rec. 2002, 1807, 51–58. [Google Scholar] [CrossRef]
- Naveh, K.S.; Kim, J. Urban trajectory analytics: Day-of-week movement pattern mining using tensor factorization. IEEE Trans. Intell. Transp. Syst. 2019, 20, 2540–2549. [Google Scholar] [CrossRef]
- Sirmatel, I.I.; Geroliminis, N. Nonlinear moving horizon estimation for large-scale urban road networks. IEEE Trans. Intell. Transp. Syst. 2020, 21, 4983–4994. [Google Scholar] [CrossRef]
- Li, D.; Yang, M.; Jin, C.-J.; Ren, G.; Liu, X.; Liu, H. Multi-modal combined route choice modeling in the MaaS age considering generalized path overlapping problem. IEEE Trans. Intell. Transp. Syst. 2021, 22, 2430–2441. [Google Scholar] [CrossRef]
- Ma, L.; Yuan, F.; Yan, X.; Zhang, J. Disentangling city-level macroscopic traffic performance patterns through a trigonometric multiseasonal filtering algorithm: Inspiration from big data of ride-sourcing trips. J. Transp. Eng. Part A Syst. 2022, 148, 04021120. [Google Scholar] [CrossRef]
- Parry, K.; Hazelton, M.L. Bayesian inference for day-to-day dynamic traffic models. Transp. Res. Part B Methodol. 2013, 50, 104–115. [Google Scholar] [CrossRef]
- Friesz, T.L.; Bernstein, D.; Stough, R. Dynamic systems, variational inequalities and control theoretic models for predicting time varying urban network flows. Transp. Sci. 1996, 30, 14–31. [Google Scholar] [CrossRef]
- Cascetta, E. A stochastic process approach to the analysis of temporal dynamics in transportation networks. Transp. Res. Part B Methodol. 1989, 23, 1–17. [Google Scholar] [CrossRef]
- Cantarella, G.; Velona, P. Stability analysis of equilibrium patterns in a transportation network. In Proceedings of the European Transport Conference (ETC), Strasbough, France, 8–10 October 2003. [Google Scholar]
- Bie, J.; Lo, H. Stability and attraction domains of traffic equilibria in a day-to-day dynamical system formulation. Transp. Res. Part B Methodol. 2010, 44, 90–107. [Google Scholar] [CrossRef]
- Li, J.; Wu, Z.; Shen, Z. Fitting and characteristics analysis of travel-time fluctuations on an urban road network. J. Transp. Eng. Part A Syst. 2021, 147, 04021058. [Google Scholar] [CrossRef]
- Hegyi, A.; de Schutter, B.; Hellendoorn, H. Model predictive control for optimal coordination of ramp metering and variable speed limits. Transp. Res. Part C Emerg. Technol. 2005, 13, 185–209. [Google Scholar] [CrossRef]
- Bellemans, T.; de Schutter, B.; de Moor, B. Model predictive control for ramp metering of motorway traffic: A case study. Control Eng. Pract. 2006, 14, 757–767. [Google Scholar] [CrossRef]
- Kotsialos, A.; Papageorgiou, M.; Mangeas, M.; Haj-Salem, H. Coordinated and integrated control of motorway networks via nonlinear optimal control. Transp. Res. Part C Emerg. Technol. 2002, 10, 65–84. [Google Scholar] [CrossRef]
- Fletcher, R.; Leyffer, S. Numerical experience with lower bounds for MIQP branch-and-bound. SIAM J. Optim. 1998, 8, 604–616. [Google Scholar] [CrossRef]
- van den Berg, M.; de Schutter, B.; Hellendoorn, J.; Hegyi, A. Influencing route choice in traffic networks: A model predictive control approach based on mixed-integer linear programming. In Proceedings of the IEEE International Conference on Control Applications, San Antonio, TX, USA, 3–5 September 2008. [Google Scholar] [CrossRef]
- van den Berg, M.; de Schutter, B.; Hegyi, A.; Hellendoorn, H. Day-to-day route choice control in traffic networks with time-varying demand profiles. In Proceedings of the European Control Conference (ECC), Budapest, Hungary, 23–26 August 2009. [Google Scholar] [CrossRef]
- Yang, H.; Huang, H. The multi-class, multi-criteria traffic network equilibrium and systems optimum problem. Transp. Res. Part B Methodol. 2004, 38, 1–15. [Google Scholar] [CrossRef]
- Wardrop, J.G. Some theoretical aspects of road traffic research. Proc. Inst. Civ. Eng. 1952, 1, 325–362. [Google Scholar] [CrossRef]
- Smith, M.J. The existence, uniqueness and stability of traffic equilibria. Transp. Res. Part B Methodol. 1979, 13, 259–304. [Google Scholar] [CrossRef]
- Dafermos, S.C. Traffic equilibrium and variational inequalities. Transp. Sci. 1980, 14, 42–54. [Google Scholar] [CrossRef]
- Josefsson, M.; Patriksson, M. Sensitivity analysis of separable traffic equilibrium equilibria with application to bilevel optimization in network design. Transp. Res. Part B Methodol. 2007, 41, 4–31. [Google Scholar] [CrossRef]
- Patriksson, M. Sensitivity analysis of traffic equilibria. Transp. Sci. 2004, 38, 258–281. [Google Scholar] [CrossRef]
- Patriksson, M.; Rockafellar, R.T. Sensitivity analysis of aggregated variational inequality problems, with application to traffic equilibria. Transp. Sci. 2003, 37, 56–58. [Google Scholar] [CrossRef]
- Tobin, R.L.; Friesz, T.L. Sensitivity analysis for equilibrium network flow. Transp. Sci. 1988, 22, 231–293. [Google Scholar] [CrossRef]
- Cho, H.J.; Smith, T.E.; Friesz, T.L. A reduction method for local sensitivity analysis of network equilibrium arc flows. Transp. Res. Part B Methodol. 2000, 34, 31–51. [Google Scholar] [CrossRef]
- Yang, H.; Bell, M.G. Sensitivity analysis of network traffic equilibrium revisited: The corrected approach. In Proceedings of the 4th IMA International Conference on Mathematic in Transport, London, UK, 7–9 September 2007. [Google Scholar]
- Wang, J.; He, X.; Peeta, S. Sensitivity analysis based approximation models for day-to-day link flow evolution process. Transp. Res. Part B Methodol. 2016, 92, 35–53. [Google Scholar] [CrossRef]
- Nakayama, S.; Watling, D. Consistent formulation of network equilibrium with stochastic flows. Transp. Res. Part B Methodol. 2014, 66, 50–69. [Google Scholar] [CrossRef]
- Sun, C.; Jing, H.; Chen, T.; Li, M.; Zhang, P. Incremental equilibrium assighment and application to traffic network model. IET Intell. Transp. Syst. 2023, 17, 794–803. [Google Scholar] [CrossRef]
- Huang, K.; Chen, M.; Zhou, Z.; Han, X.; Wang, J.; Liu, Z. A systematic approach for the calibration of route choice models based on stochastic user equilibrium. IEEE Intell. Transp. Syst. Mag. 2023, 15, 55–71. [Google Scholar] [CrossRef]
- Site, P.D. Fixed-point states of day-to-day assignment processes with state-dependent route choice. Transp. Res. Procedia 2017, 27, 1009–1016. [Google Scholar] [CrossRef]
- Guo, X.; Liu, H.X. Bounded rationality and irreversible network change. Transp. Res. Part B Methodol. 2011, 45, 1606–1618. [Google Scholar] [CrossRef]
- Guo, X. Toll sequence operation to realize target flow pattern under bounded rationality. Transp. Res. Part B Methodol. 2013, 56, 203–216. [Google Scholar] [CrossRef]
- Torkjazi, M.; Mirjafari, P.S.; Poorzahedy, H. Reliability-based network flow estimation with day-to-day variation: A model validation on real large-scale urban networks. J. Intell. Transp. Syst. 2018, 22, 121–143. [Google Scholar] [CrossRef]
- Guo, Z.; Wang, D.Z.W.; Wang, D. Managing mixed traffic with autonomous vehicles—A day-to-day routing allocation scheme. Transp. Res. Part C Emerg. Technol. 2022, 140, 103726. [Google Scholar] [CrossRef]
- Sun, M. A day-to-day dynamic model for mixed traffic flow of autonomous vehicles and inertial human-driven vehicles. Transp. Res. Part E Logist. Transp. Rev. 2023, 173, 103113. [Google Scholar] [CrossRef]
- Liang, Q.; Li, X.; Chen, Z.; Pan, T.; Zhong, R. Day-to-day traffic control for networks mixed with regular human-piloted and connected autonomous vehicles. Transp. Res. Part B Methodol. 2023, 178, 102847. [Google Scholar] [CrossRef]
- Haj-Salem, H.; Papageorgiou, M. Ramp metering impact on urban corridor traffic: Field results. Transp. Res. Part A Policy Pract. 1995, 29, 303–319. [Google Scholar] [CrossRef]
- Iida, Y.; Akiyama, T.; Uchida, T. Experimental analysis of dynamic route choice behavior. Transp. Res. Part B Methodol. 1992, 26, 17–32. [Google Scholar] [CrossRef]
- Poulydoropoulou, A.; Ben-Akiva, M.; Kaysi, I. Influence of traffic information on drivers’ route choice behavior. Transp. Res. Rec. 1994, 1453, 56–65. [Google Scholar]
- Jiang, G.; Zheng, Z.; Bai, Z.; Dai, L.; Zhao, J. Simulation-based assessment of variable message signs route guidance information under congestion condition. J. Jilin Univ. (Eng. Technol. Ed.) 2006, 36, 183–187. [Google Scholar] [CrossRef]
- Klügl, F.; Bazzan, A.L.C. Simulation studies on adaptive route decision and the influence of information on commuter scenarios. J. Intell. Transp. Syst. 2004, 8, 223–232. [Google Scholar] [CrossRef]
- Cantarella, G.E. Day-to-day dynamic models for intelligent transportation systems design and appraisal. Transp. Res. Part C Emerg. Tecnol. 2013, 29, 117–130. [Google Scholar] [CrossRef]
- Roy, T.; Dey, S. Route choice-based socio-technical macroscopic traffic model. IEEE Trans. Intell. Transp. Syst. 2023, 24, 5781–5791. [Google Scholar] [CrossRef]
- He, X.; Guo, X.; Liu, H.X. A link-based day-to-day traffic assignment model. Transp. Res. Part B Methodol. 2010, 44, 597–608. [Google Scholar] [CrossRef]
- Guo, R.Y.; Yang, H.; Huang, H.J. A discrete rational adjustment process of link flows in traffic networks. Transp. Res. Part C Emerg. Technol. 2013, 34, 121–137. [Google Scholar] [CrossRef]
- Guo, R.Y.; Yang, H.; Huang, H.J.; Tan, Z. Link-based day-to-day network traffic dynamics and equilibria. Transp. Res. Part B Methodol. 2015, 71, 248–260. [Google Scholar] [CrossRef]
- Han, L.; Du, L. On a link-based day-to-day traffic assignment model. Transp. Res. Part B Methodol. 2012, 46, 72–84. [Google Scholar] [CrossRef]
- Smith, M.; Mounce, R. A splitting rate model of traffic re-routing and traffic control. Transp. Res. Part B Methodol. 2011, 45, 1389–1409. [Google Scholar] [CrossRef]
- Zhu, Z.; Mardan, A.; Zhu, S.; Yang, H. Capturing the interaction between travel time reliability and route choice behavior based on the generalized Bayesian traffic model. Transp. Res. Part B Methodol. 2021, 143, 48–64. [Google Scholar] [CrossRef]
- Yang, H.; Huang, H. Principle of marginal-cost pricing: How does it work in a general network? Transp. Res. Part A Policy Pract. 1998, 32, 45–54. [Google Scholar] [CrossRef]
- Henderson, J. Road congestion: A reconsideration of pricing theory. J. Urban Econ. 1974, 1, 346–365. [Google Scholar] [CrossRef]
- Carey, M.; Srinivasan, A. Externalities, average and marginal costs, and tolls on congested networks with time-varying flows. Oper. Res. 1993, 41, 217–231. [Google Scholar] [CrossRef]
- Yang, H.; Meng, Q. Departure time, route choice and congestion toll in a queuing network with elastic demand. Transp. Res. Part B Methodol. 1998, 32, 247–260. [Google Scholar] [CrossRef]
- Wie, B. Dynamic Stackelberg equilibrium congestion pricing. Transp. Res. Part C Emerg. Technol. 2007, 15, 154–174. [Google Scholar] [CrossRef]
- Sandholm, W.H. Evolutionary implementation and congestion pricing. Rev. Econ. Stud. 2002, 69, 667–689. [Google Scholar] [CrossRef]
- Friesz, T.L.; Bernstein, D.; Kydes, N. Dynamic congestion pricing in disequilibrium. Netw. Spat. Econ. 2004, 4, 181–202. [Google Scholar] [CrossRef]
- Yang, F.; Yin, Y.; Lu, J. Steepest descent day-to-day dynamic toll. Transp. Res. Rec. 2007, 2039, 83–90. [Google Scholar] [CrossRef]
- Tan, Z.; Yang, H.; Guo, R. Dynamic congestion pricing with day-to-day flow evolution and user heterogeneity. Transp. Res. Part C Emerg. Technol. 2015, 61, 87–105. [Google Scholar] [CrossRef]
- Irfan, M.; Khurshid, A.N.; Khurshid, M.B.; Ali, Y.; Khattak, A. Policy implications of work-trip mode choice using econometric modeling. J. Transp. Eng. Part A Syst. 2018, 144, 04018035. [Google Scholar] [CrossRef]
- Zhang, W.; Zhang, H.M.; Guan, W.; Yan, X. Managing day-to-day network traffic evolution via an altering ex-post information release strategy. J. Transp. Eng. Part A Syst. 2018, 144, 04018028. [Google Scholar] [CrossRef]
- Liu, Z.; Wang, X.; Dai, J.; Li, X.; Li, R. Impacts of COVID-19 pandemic on travel behavior in large cities of china: Investigation on the lockdown and reopening phases. J. Transp. Eng. Part A Syst. 2022, 148, 05021011. [Google Scholar] [CrossRef]
- He, X.; Liu, H.X. Modeling the day-to-day traffic evolution process after an unexpected network disruption. Transp. Res. Part B Methodol. 2012, 46, 50–71. [Google Scholar] [CrossRef]
- Marra, A.D.; Corman, F. How different network disturbances affect route choice of public transport passengers. A descriptive study based on tracking. Expert Syst. Appl. 2023, 213, 119083. [Google Scholar] [CrossRef]
- Barroso, J.M.F.; Albuquerque-Oliveira, J.L.; Oliveira-Neto, F.M. Correlation analysis of day-to-day origin-destination flows and traffic volumes in urban networks. J. Transp. Geogr. 2020, 90, 102899. [Google Scholar] [CrossRef]
- Tang, M. The Simulation System of Individual Travel Behavior Based on Swarm. Master’s Thesis, Tianjin University, Tianjin, China, 2008. [Google Scholar]
- Yang, C.; Ye, W.; Zhu, R.; Zhang, T. Resident activity pattern recognition and comparison of six Sino-American metropolises. IET Intell. Transp. Syst. 2019, 13, 443–452. [Google Scholar] [CrossRef]
- Qi, G.; Huang, A.; Guan, W.; Fan, L. Analysis and prediction of regional mobility pattern of bus travellers using smart card data and points of interest data. IEEE Trans. Intell. Transp. Syst. 2019, 20, 1197–1204. [Google Scholar] [CrossRef]
- Zhao, X.; Zhang, Y.; Hu, Y.; Wang, S.; Li, Y.; Qian, S.; Yin, B. Interactive visual exploration of human mobility correlation based on smart card data. IEEE Trans. Intell. Transp. Syst. 2021, 22, 4825–4837. [Google Scholar] [CrossRef]
- Zeng, Z.; Yi, W.; Wang, S.; Qu, X. Emergency vehicle routing in urban road networks with multistakeholder cooperation. J. Transp. Eng. Part A Syst. 2021, 147, 04021064. [Google Scholar] [CrossRef]
- Fekih, M.; Bonnetain, L.; Furno, A.; Bonnel, P.; Smoreda, Z.; Galland, S.; Bellemans, T. Potential of cellular signaling data for time-of-day estimation and spatial classification of travel demand: A large-scale comparative study with travel survey and land use data. Transp. Lett. 2022, 14, 787–805. [Google Scholar] [CrossRef]
- Barmpounakis, E.; Vlahogianni, E.I. Powered two-wheeler detection using crowdsourced smartphone data. IEEE Trans. Intell. Veh. 2020, 5, 575–584. [Google Scholar] [CrossRef]
- Vial, A.; Daamen, W.; Ding, A.Y.; van Arem, B.; Hoogendoorn, S. AMSense: How mobile sensing platforms capture pedestrian/cyclist spatiotemporal properties in cities. IEEE Intell. Transp. Syst. Mag. 2020, 14, 29–43. [Google Scholar] [CrossRef]
- Yao, Y.; Zhang, H.; Chen, J.; Li, W.; Shibasake, R.; Song, X. Mobility tableau: Human mobility similarity measurement for city dynamics. IEEE Trans. Intell. Transp. Syst. 2023, 24, 7108–7121. [Google Scholar] [CrossRef]
- Guo, Y.; Yang, F.; Yan, H.; Xie, S.; Liu, H.; Dai, Z. Activity-based model based on multi-day cellular data: Considering the lack of personal attribute and activity type. IET Intell. Transp. Syst. 2023, 17, 2474–2492. [Google Scholar] [CrossRef]
- Huang, Y.; Wang, D.; Xu, W.; Cai, Z.; Fu, F. Accurate map matching method for mobile phone signaling data under spatio-temporal uncertainty. IEEE Trans. Intell. Transp. Syst. 2024, 25, 1418–1429. [Google Scholar] [CrossRef]
- Xu, X.; Qu, K.; Chen, A.; Yang, C. A new day-to-day dynamic network vulnerability analysis approach with Weibit-based route adjustment process. Transp. Res. Part E Logist. Transp. Rev. 2021, 153, 102421. [Google Scholar] [CrossRef]
- Li, W.; Ma, S.; Jia, N.; He, Z. An analyzable agent-based framework for modeling day-to-day route choice. Transp. A Transp. Sci. 2022, 18, 1517–1543. [Google Scholar] [CrossRef]
- Meneguzzer, C. Modeling the combined effect of travelers’ contrarian behavior, learning and inertia on the day-to-day dynamics of route choice. Appl. Sci. 2023, 13, 3294. [Google Scholar] [CrossRef]
- Zhu, Z.; Zhu, S.; Sun, L.; Mardan, A. Modelling changes in travel behaviour mechanisms through a high-order hidden Markov model. Transp. A Transp. Sci. 2024, 20, 2130731. [Google Scholar] [CrossRef]
- Guarda, P.; Battifarano, M.; Qian, S. Estimating network flow and travel behavior using day-to-day system-level data: A computational graph approach. Transp. Res. Part C Emerg. Technol. 2024, 158, 104409. [Google Scholar] [CrossRef]
- Zhang, R.; Xiong, J.; Huang, Y.; Sumalee, A.; Chow, A.H.F.; Pan, T. Dynamic system optimum analysis of multi-region macroscopic fundamental diagram systems with state-dependent time-varying delays. IEEE Trans. Intell. Transp. Syst. 2020, 21, 4000–4016. [Google Scholar] [CrossRef]
- Kazhamiakin, R.; Loria, E.; Moaconi, A.; Scanagatta, M. A gamification platform to analyze and influence citizens’ daily transportation choices. IEEE Trans. Intell. Transp. Syst. 2021, 22, 2153–2167. [Google Scholar] [CrossRef]
- Zhang, P.; Lei, F.; Ma, Y.; Liu, L.; Zhu, G. The general system model of vehicle TDM effect on urban road traffic. IEEE Intell. Transp. Syst. Mag. 2021, 13, 160–168. [Google Scholar] [CrossRef]
- Zhang, P.; Ma, W.; Qian, S. Cluster analysis of day-to-day traffic data in networks. Transp. Res. Part C Emerg. Technol. 2022, 144, 103882. [Google Scholar] [CrossRef]
- Xiao, Z.; Li, H.; Jiang, H.; Li, Y.; Alazab, M.; Zhu, Y.; Dustdar, S. Predicting urban region heat via learning arrive-stay-leave behaviors of private cars. IEEE Trans. Intell. Transp. Syst. 2023, 24, 10843–10856. [Google Scholar] [CrossRef]
- Vitetta, A. A quantum utility model for route choice in transport systems. Travel Behav. Soc. 2016, 3, 29–37. [Google Scholar] [CrossRef]
- Lipovetsky, S. Quantum paradigm of probability amplitude and complex utility in entangled discrete choice modeling. J. Choice Model. 2018, 27, 62–73. [Google Scholar] [CrossRef]
- Wang, S.; Pei, Z.; Wang, C.; Wu, J. Shaping the future of the application of quantum computing in intelligent transportation system. Intell. Converg. Netw. 2021, 2, 259–276. [Google Scholar] [CrossRef]
- Cooper, C.H.V. Exploring potential applications of quantum computing in transportation modeling. IEEE Trans. Intell. Transp. Syst. 2022, 23, 14712–14720. [Google Scholar] [CrossRef]
- Zhao, C.; Wang, Y.; Wu, H.; Sun, Y.; Ding, L. Experimental study on quantum decision-making of travel choice behavior. J. Transp. Eng. Inf. 2023, 21, 29–41. [Google Scholar] [CrossRef]
Figure 1.
The overall framework of this review.
Figure 1.
The overall framework of this review.
Figure 2.
Process abstract of micro travel decision-making.
Figure 2.
Process abstract of micro travel decision-making.
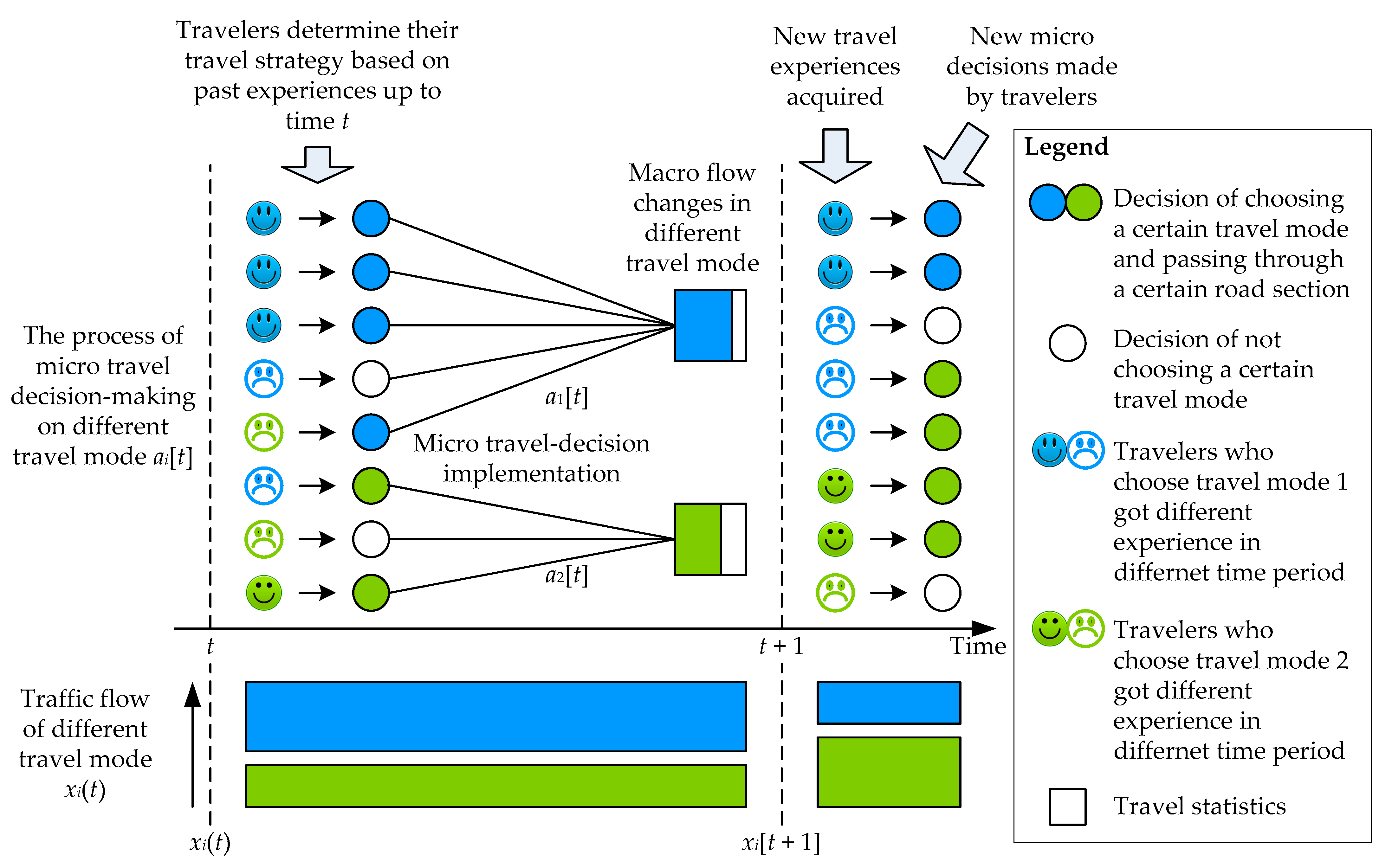
Table 1.
The list of abbreviations that appear in this review.
Table 1.
The list of abbreviations that appear in this review.
Abbreviation | Definition | Abbreviation | Definition |
---|---|---|---|
ABM | Activity-based model | ARCHs | Autoregressive conditional heteroskedasticity family models |
ART | Approximate reasoning for transportation | ASL | Arrive-stay-leave |
ATC | Automatic toll collection | ATIS | Advanced traveler information system |
AV | Autonomous vehicle | BDI | Beliefs, desires and intentions |
BRUE | Bounded rationality user equilibrium | CCL | Congestion-based conditional Logit |
FCD | Floating car data | FVP | Frequently visited point |
GPS | Global positioning system | HMM | Hidden Markov model |
HV | Human-driven vehicle | ICSS | Iterative cumulative sums of squares |
ICT | Information and communication technology | IRL | Inverse reinforcement learning |
ITS | Intelligent transportation system | LBS | Location-based service |
LCL | Length-based conditional Logit | LRP | Linear rewards and punishments |
MFD | Macroscopic fundamental diagram | MFG | Mean field game |
MILP | Mixed-integer linear programming | MNL | Multinomial Logit |
MPC | Model predictive control | MSD | Mobile signaling data |
NMHE | Nonlinear moving horizon estimation | NP | Non-deterministic polynomial |
OD | Origin and destination | PUE | Pessimistic user equilibrium |
RAP | Resident activity pattern | rePRAP | Relative proportion-based route adjustment process |
RGS | Route guidance system | RL | Reinforcement learning |
RP | Revealed preference | RTW | Return to work |
RUE | Rationality user equilibrium | RUM | Random utility model |
SCD | Smart card data | SDSUE | State-dependent stochastic user equilibrium |
SLA | Stochastic learning automata | SP | Stated preference |
SUE | Stochastic user equilibrium | TDM | Travel demand management |
TTV | Travel time volatility | UE | User equilibrium |
VI | Variational inequality | VMS | Variable message sign |
Table 2.
Comparison of deterministic and stochastic models for micro travel decision-making.
Table 2.
Comparison of deterministic and stochastic models for micro travel decision-making.
Model Types | Models | Maturity | Model Complexity |
Closeness to Real Travel States |
---|---|---|---|---|
Deterministic models | Gravity flow model [25] | In 1980s | ★ | ★ |
Percentage conversion models [26,27,28,29,30,31,32,33] | In 1980s | ★ | ★ | |
Network trial and error models [34,35,36] | In 1990s | ★★ | ★★ | |
Projection dynamic system models [37,38,39] | In 1990s | ★★ | ★★ | |
Evolutionary traffic dynamic system models [40,41,42] | In 1990s | ★★★ | ★★★ | |
Stochastic models | RUM [43] | In 1990s | ★★ | ★★ |
Stochastic learning models [44,45] | In 2000s | ★★★ | ★★★ | |
Markov random state transition theory-based models [46,47] | In 2000s | ★★★ | ★★★ | |
RL-based models [48,49,50] | In 2010s | ★★★★ | ★★★★ |
Table 3.
Research on micro travel decision-making with different information factors considered.
Table 3.
Research on micro travel decision-making with different information factors considered.
Detailed Models | Literature | Information Factors Considered | ||||
---|---|---|---|---|---|---|
Past Travel Experiences | Personal Habits |
Time Cost | Money Cost | Crowding or Congestion Level |
||
Utility theory-based models | [53] | √ | ||||
[54,55,56,57,58,59,60,61] | √ | √ | ||||
SP- and RP-based models | [55,62] | √ | ||||
[63] | √ | √ | ||||
Multi-agent- and RL-based models | [64,65] | √ | √ | √ | ||
[66] | √ | √ | ||||
[67] | √ | √ | ||||
[68] | √ | √ | ||||
[69] | √ | √ | √ | |||
Empirical models | [70,71] | √ | √ | |||
[72,73,74] | √ | |||||
[75] | √ | √ | √ | √ |
Table 4.
Research on micro decision-making of urban day-to-day travelers under the impact of an information environment.
Table 4.
Research on micro decision-making of urban day-to-day travelers under the impact of an information environment.
Research Attitudes | From the Perspective of Traffic Information | From the Perspective of Travelers |
---|---|---|
Positive | Improve urban transportation conditions [76,77,78] |
Willing to accept information prompts [79,80,81] |
Provide travel decision-making assistance [35,82,83,84,85] |
Willing to face risk with the help of information [86] |
|
Reduce emissions, protect environment [83] |
Information provided by mobile devices affects most [87] | |
Negative | Bad effects of improper information dissemination [88] | Unwilling to accept information prompts [89,90,91,92,93,94] |
Real road network information may not lead to best traffic distribution [95] | ||
Information prompts do not lead to traffic condition improvement [96] | A majority of travelers are indifferent to information prompts [97,98,99] |
|
Better to follow intuition than follow information prompts [100] | ||
Neutral | Interaction in social platforms is also a part of travel information [101,102] | Different attitudes towards information prompts [103,104,105,106] |
Information prompts may not always have a fixed effect [107,108] | ||
Effectiveness varies depending on the penetration rate of ATIS [109,110] | ||
Information dissemination has different strategies [111] | Differentiated information dissemination considering different personalities of receivers [112] |
|
Correct and incorrect information may both have good effects [113] | ||
Different impact of information prompts inside/outside congestion area [114] |
Table 5.
Research on traditional explanations for macro road traffic flow.
Table 5.
Research on traditional explanations for macro road traffic flow.
Research Objects | Topic Types | Detailed Research Concerns | Literature |
---|---|---|---|
Explanations for macro road traffic flow in urban day-to-day travel | Qualitative Macro phenomena | Complexity of macro phenomena | [118] |
Micro influencing factor: Humans | [119] | ||
Macro influencing factor: Road flow conditions | [120,121] | ||
New data acquisition methods for analyzing macro phenomena | [122,123,124,125] | ||
Quantitative Macro Traffic Flow Explanations |
Analysis of traffic flow changes caused by micro behavioral characteristics of travelers (often based on discrete time series analysis) |
[126,127,128,129,130,131] | |
Analysis of macro traffic flow adjustment and control (often based on model predictive control (MPC) and mixed-integer linear programming (MILP)) |
[132,133,134,135,136,137] |
Table 6.
Research on UE of macro traffic networks with different information factors considered.
Table 6.
Research on UE of macro traffic networks with different information factors considered.
Detailed Models | Literature | Information Factors Considered | ||||
---|---|---|---|---|---|---|
Flow on Travel Route | Flow on Travel Road Segments | Individual Travel Time Cost | Road Network Constraints | Past Road Network Status | ||
UE | [77] | √ | ||||
[78] | √ | √ | ||||
[82,83] | √ | √ | ||||
SUE | [35,84,100] | √ | √ | |||
[101] | √ | √ | √ | |||
SDSUE | [95] | √ | √ | √ | ||
RUE | [79,85] | √ | √ | √ | ||
PUE | [96] | √ | √ | |||
BRUE | [102] | √ | √ | √ |
Table 7.
Two different types of traffic information that can be obtained.
Table 7.
Two different types of traffic information that can be obtained.
Information Types | Specific Information | Literature |
---|---|---|
Global traffic information | Congestion pricing information | [172,173,174,175,176,177,178,179,180,181] |
Travel time cost information | [182] | |
Guide information after pandemic | [183] | |
Local traffic information | Local emergencies in urban road network | [184,185] |
Local congestion caused by temporary traffic accidents or commercial activities | [186] | |
Shared travel strategy between travelers | [187,188,189,190] | |
Inertial information from certain vehicle operation organizations | [191] |
Disclaimer/Publisher’s Note: The statements, opinions and data contained in all publications are solely those of the individual author(s) and contributor(s) and not of MDPI and/or the editor(s). MDPI and/or the editor(s) disclaim responsibility for any injury to people or property resulting from any ideas, methods, instructions or products referred to in the content. |
[ad_2]