Effect of Environmental and Socioeconomic Factors on Increased Early Childhood Blood Lead Levels: A Case Study in Chicago
[ad_1]
1. Introduction
2. Data
2.1. Blood Lead Levels (BLLs) and Lead Poisoning (LP) Data
2.2. Socioeconomic Data
2.3. Land Surface Temperature Data
An inherent limitation of satellite-measured LST products is their inability to capture surface temperatures under cloudy conditions. To address this limitation, we refined our dataset by exclusively selecting observations recorded during hours with less than 10% cloud cover within our study area. This selection criterion ensures that we utilized a subset of data that can be reliably considered cloud-free. As a result of this filtering process, we obtained 7724 h of usable LST data from 2019 to 2021, constituting approximately 30% of the total available temperature data. This subset is adequate for our analysis as our primary interest lies in examining the relative temperature differences across zip codes rather than the absolute magnitude of LST.
As a secondary step in our analysis, we calculated the LST anomaly for each of the 7724 time steps. For each grid point within the 2 km resolution data, we subtracted the mean LST of the entire study area at the corresponding time step to determine relative temperature differences. This calculation produced 7724 spatial anomaly maps.
2.4. Interrelationships among Predictors
3. Method of Analysis
In our approach, a spline function is fitted for each predictor variable, utilizing a basis of 12 cubic splines. This configuration allows the GAM to produce a smooth curve that accurately reflects the relationship between each predictor and the dependent variable. Opting for 12 cubic splines grants the model ample flexibility to capture complex, non-linear patterns effectively. To enhance the fidelity and generalization ability of these spline functions, smoothing parameter (λ) values are meticulously adjusted for each predictor. These λ values play a pivotal role in modulating the smoothness of the spline functions. To determine the optimal λ values, we employed a grid search algorithm by testing a spectrum of λ values to pinpoint the one that minimizes the root mean squared error (RMSE) of the model’s predictions, thereby ensuring a precise and reliable prediction of LP rate.
4. Results
4.1. Wilcoxon Signed-Rank Test for Individual Predictors
4.2. LP Rate Modelling with GAM
The analysis of daytime and nighttime LST reveals detailed differences in their relationships with LP. While both temperatures correlate positively with LP, the influence of daytime LST plateaus after a 1 °C anomaly, suggesting a threshold effect. Conversely, nighttime LST demonstrates a steady positive relationship with LP, with higher confidence levels than its daytime counterpart. This distinction may be attributed to the better representation of urban fabric by nighttime LST, which captures the retained heat within built environments more effectively than daytime LST.
4.3. Racial Inequality
Until this point, the analysis has concentrated on per capita income, unemployment rate, building age, and LST, without integrating racial demographics for each block. This approach is because, within the Chicagoland context, racial demographics exhibit a high correlation with the aforementioned socioeconomic and environmental metrics, suggesting that including racial data directly does not significantly enhance the predictive capability of our model. Nonetheless, the GAM framework allows for an exploration of how LP rates may be differentially influenced by socioeconomic status across various racial groups within zip codes.
To investigate the influence of socioeconomic factors on LP rates within different racial groups, we initially identified zip codes predominantly inhabited by specific racial demographics—White, Black, and Hispanic/Latino (HisLat)—based on the criterion that the majority population (over 50%) of the zip code belongs to one of these racial categories according to the Census data. Subsequently, we compiled the values of each predictor variable (per capita income, unemployment rate, education rate, building age, daytime LST, and nighttime LST) for the zip codes categorized by these racial demographics.
The underlying factors contributing to this disparity differ between the two groups. For the HisLat population, the majority of the increased LP rate is attributable to building age, which accounts for 82% of the HisLat-specific increase and 1.23% absolute increase in LP rate. In contrast, for the Black population, the increased LP rate is largely due to both the unemployment rate—contributing to 50% of the Black-specific increase and a 0.73% increase in LP rate—and building age, which accounts for 40% of the increase and a 0.58% absolute rise in LP rate.
While our modelling-based analysis identifies socioeconomic factors and building age as key contributors to LP rate disparities, it is essential to recognize these factors within a broader historical and social context. Historical housing policies, particularly redlining, have ingrained systemic racial discrimination into urban landscapes, influencing residential patterns and health outcomes to this day. These policies have not only marginalized certain communities through economic constraints but have also exposed them to greater environmental risks, including deteriorated housing and proximity to pollutants.
5. Summary and Conclusions
In this study, we investigated the percentage of children with blood lead levels (BLLs) exceeding 5 µg/dL across zip codes in the Chicagoland area from 2019 to 2021, examining the association with socioeconomic and environmental factors and the broader context of racial inequality.
Moreover, our study analyzes the relationship between satellite-based measurements of land surface temperature (LST) variations during daytime and nighttime and their impact on LP rate, a previously unexplored area. We hypothesize that higher LSTs may contribute to increased LP rates by enhancing the solubility of lead in antiquated plumbing systems, thus elevating the potential for lead to leach into the drinking water supply within communities living within an older infrastructure. Our findings indicate that both daytime and nighttime LST are associated with increased LP, with a more marked effect observed for nighttime LST. The heightened impact of nighttime LST likely stems from its effectiveness as an indicator of the urban heat island effect, which captures the thermal retention properties of urban structures and surfaces. Nighttime LST is particularly indicative of the heat generated and retained by built environments, which can have various indirect effects on LP. For instance, higher nighttime LST may be reflective of less vegetated areas or denser building materials, both of which are factors that can indirectly contribute to elevated LP rate. This aspect of the study not only extends the understanding of environmental influences on LP rates but also underscores the potential of satellite-derived LST data as a valuable tool in public health research.
Lastly, our investigation addresses racial disparities in LP rates. We find that Black and Hispanic/Latino communities are at risk from an elevated LP rate. The factors contributing to this increased exposure differ somewhat between groups. For the Black population, the heightened risk is primarily due to the high unemployment rates and the prevalence of older housing. In contrast, for Hispanic/Latino communities, the risk is predominantly associated with the presence of older housing stock. This analysis underscores a critical public health challenge: socioeconomically disadvantaged communities, particularly Black and Hispanic/Latino populations, are disproportionately affected by LP. The compounded issue of higher LP rates and limited access to mitigating resources highlights the need for dedicated public health initiatives. There is evident demand for targeted interventions and supportive measures that are tailored to address the distinct challenges faced by these vulnerable groups. Such initiatives are essential not only to alleviate the immediate burden of LP but also to foster long-term health and wellbeing within these communities. Also, the observed higher rates LP within minority populations merit attention, particularly considering how stressors associated with poverty, racism, and stereotype threats prevalent among these groups could influence the body’s biotransformation processes. Such factors might contribute to the disparities in LP rates observed across different demographic groups.
This study is notable for several reasons. Firstly, it utilizes recent data from 2019 to 2021, providing a contemporary analysis of the factors contributing to LP. Secondly, it extends beyond the urban center to encompass the wider Chicagoland area, offering insights into a broader demographic. Thirdly, satellite-based measurements of LST are employed, highlighting the value of remotely sensed environmental data in public health research and further highlighting the possible increase in LP in a warming world. Fourthly, advanced statistical modeling through GAM is applied to understand the non-linear effects of socioeconomic and environmental variables on LP rates. Lastly, the study addresses racial disparities by examining how socioeconomic conditions correlated with race indirectly affect LP rates, providing an informed perspective on this complex issue.
6. Limitation and Future Direction
It is important to acknowledge that while our regression model did not reveal a significant correlation between LP and educational factors, this could be due to the high correlation between education levels and other variables, such as income and unemployment rates, which have demonstrated strong associations with LP. Additionally, the lack of observed significance may be attributed to the spatial and temporal scales considered in this study.
Furthermore, the dataset covers a relatively short period of just three years. With a more extended dataset, it would be possible to examine the general trends or zip-code-specific trends in LP rates over time, assessing not just the disparities between zip codes but also how they evolve. Additionally, other environmental factors, such as wind speed or humidity, could potentially enhance the analysis. However, obtaining these variables at a high spatial resolution is challenging, as they are not readily measurable via satellite technologies.
Author Contributions
Conceptualization, J.L. and M.H.; data curation, J.L. and M.H.; formal analysis, J.L. and M.H.; funding acquisition, J.L.; investigation, J.L. and M.H.; methodology, J.L. and M.H.; resources, J.L. and M.H.; validation, J.L.; visualization, J.L.; writing—original draft, J.L., writing—review and editing, J.L. and M.H. All authors have read and agreed to the published version of the manuscript.
Funding
This material is based upon work supported by the U.S. Department of Energy, Office of Science, Office of Biological and Environmental Research’s Urban Integrated Field Laboratories CROCUS project research activity, under Award Number DE-SC0023226.
Institutional Review Board Statement
Not applicable.
Informed Consent Statement
Not applicable.
Data Availability Statement
Conflicts of Interest
The authors declare no conflicts of interest.
References
- Bellinger, D.; Needleman, H.; Eden, A.; Donohoe, M.; Canfield, R.; Henderson, C. Intellectual impairment and blood lead levels. N. Engl. J. Med. 2003, 349, 500–502. [Google Scholar]
- Bellinger, D.C.; Stiles, K.M.; Needleman, H.L. Low-level lead exposure, intelligence and academic achievement: A long-term follow-up study. Pediatrics 1992, 90, 855–861. [Google Scholar] [CrossRef]
- Naranjo, V.I.; Hendricks, M.; Jones, K.S. Lead toxicity in children: An unremitting public health problem. Pediatr. Neurol. 2020, 113, 51–55. [Google Scholar] [CrossRef] [PubMed]
- Hauptman, M.; Bruccoleri, R.; Woolf, A.D. An update on childhood lead poisoning. Clin. Pediatr. Emerg. Med. 2017, 18, 181–192. [Google Scholar] [CrossRef] [PubMed]
- WHO. Childhood Lead Poisoning; WHO: Geneva, Switzerland, 2010. [Google Scholar]
- Njati, S.Y.; Maguta, M.M. Lead-based paints and children’s PVC toys are potential sources of domestic lead poisoning–A review. Environ. Pollut. 2019, 249, 1091–1105. [Google Scholar] [CrossRef] [PubMed]
- Ara, A.; Usmani, J.A. Lead toxicity: A review. Interdiscip. Toxicol. 2015, 8, 55–64. [Google Scholar]
- Hanna-Attisha, M.; LaChance, J.; Sadler, R.C.; Champney Schnepp, A. Elevated blood lead levels in children associated with the Flint drinking water crisis: A spatial analysis of risk and public health response. Am. J. Public Health 2016, 106, 283–290. [Google Scholar] [CrossRef] [PubMed]
- Rio, M.D.; Gibson, J.M. Factors influencing inequities in lead exposure in United States children: A systematic review. Int. Public Health J. 2023, 15, 263. [Google Scholar]
- Leech, T.G.; Adams, E.A.; Weathers, T.D.; Staten, L.K.; Filippelli, G.M. Inequitable chronic lead exposure. Fam. Community Health 2016, 39, 151–159. [Google Scholar] [CrossRef] [PubMed]
- Brown, M.J.; Gardner, J.; Sargent, J.D.; Swartz, K.; Hu, H.; Timperi, R. The effectiveness of housing policies in reducing children’s lead exposure. Am. J. Public Health 2001, 91, 621. [Google Scholar]
- Brown, M.J.; Margolis, S. Lead in drinking water and human blood lead levels in the United States. Cent. Dis. Control. Prev. 2012, 61, 1–9. [Google Scholar]
- Sampson, R.J.; Winter, A.S. The racial ecology of lead poisoning: Toxic inequality in Chicago neighborhoods, 1995–2013. Du Bois Rev. Soc. Sci. Res. Race 2016, 13, 261–283. [Google Scholar] [CrossRef]
- Evens, A.; Hryhorczuk, D.; Lanphear, B.P.; Rankin, K.M.; Lewis, D.A.; Forst, L.; Rosenberg, D. The impact of low-level lead toxicity on school performance among children in the Chicago Public Schools: A population-based retrospective cohort study. Environ. Health 2015, 14, 21. [Google Scholar] [CrossRef] [PubMed]
- Potash, E.; Brew, J.; Loewi, A.; Majumdar, S.; Reece, A.; Walsh, J.; Rozier, E.; Jorgenson, E.; Mansour, R.; Ghani, R. Predictive modeling for public health: Preventing childhood lead poisoning. In Proceedings of the 21th ACM SIGKDD International Conference on Knowledge Discovery and Data Mining, Sydney, Australia, 10–13 August 2015; pp. 2039–2047. [Google Scholar]
- Oyana, T.J.; Margai, F.M. Spatial patterns and health disparities in pediatric lead exposure in Chicago: Characteristics and profiles of high-risk neighborhoods. Prof. Geogr. 2010, 62, 46–65. [Google Scholar] [CrossRef]
- Witzling, L.; Wander, M.; Phillips, E. Testing and educating on urban soil lead: A case of Chicago community gardens. J. Agric. Food Syst. Community Dev. 2010, 1, 167–185. [Google Scholar] [CrossRef]
- Laidlaw, M.A.; Zahran, S.; Mielke, H.W.; Taylor, M.P.; Filippelli, G.M. Re-suspension of lead contaminated urban soil as a dominant source of atmospheric lead in Birmingham, Chicago, Detroit and Pittsburgh, USA. Atmos. Environ. 2012, 49, 302–310. [Google Scholar] [CrossRef]
- White, D.; Gala, T. Environmental injustice? Disparities in the exposure to environmental lead poisoning and risks among children in the Chicago Neighborhoods. Am. J. Public Health 2022, 10, 124–133. [Google Scholar] [CrossRef]
- Tang, Z.; Carrel, M. The more things change the more they stay the same: Persistent spatial inequity in pediatric lead poisoning in Chicago. Appl. Geogr. 2022, 142, 102686. [Google Scholar] [CrossRef]
- Reyes, N.L.; Wong, L.-Y.; MacRoy, P.M.; Curtis, G.; Meyer, P.A.; Evens, A.; Brown, M.J. Identifying housing that poisons: A critical step in eliminating childhood lead poisoning. J. Public Health Manag. Pract. 2006, 12, 563–569. [Google Scholar] [CrossRef]
- Mayans, L. Lead poisoning in children. Am. Fam. Physician 2019, 100, 24–30. [Google Scholar]
- Yiin, L.-M.; Rhoads, G.G.; Lioy, P.J. Seasonal influences on childhood lead exposure. Environ. Health Perspect. 2000, 108, 177–182. [Google Scholar] [CrossRef]
- Chapman, S.; Watson, J.E.; Salazar, A.; Thatcher, M.; McAlpine, C.A. The impact of urbanization and climate change on urban temperatures: A systematic review. Landsc. Ecol. 2017, 32, 1921–1935. [Google Scholar] [CrossRef]
- Argüeso, D.; Evans, J.P.; Fita, L.; Bormann, K.J. Temperature response to future urbanization and climate change. Clim. Dyn. 2014, 42, 2183–2199. [Google Scholar] [CrossRef]
- Sachindra, D.; Ng, A.; Muthukumaran, S.; Perera, B. Impact of climate change on urban heat island effect and extreme temperatures: A case-study. Q. J. R. Meteorol. Soc. 2016, 142, 172–186. [Google Scholar] [CrossRef]
- Whitehead, L.S.; Buchanan, S.D. Childhood lead poisoning: A perpetual environmental justice issue? J. Public Health Manag. Pract. 2019, 25, S115–S120. [Google Scholar] [CrossRef]
- LeBrón, A.M.; Torres, I.R.; Valencia, E.; Dominguez, M.L.; Garcia-Sanchez, D.G.; Logue, M.D.; Wu, J. The state of public health lead policies: Implications for urban health inequities and recommendations for health equity. Int. J. Environ. Res. Public Health 2019, 16, 1064. [Google Scholar] [CrossRef]
- Ferrie, J.; Rolf, K.; Troesken, W. Lead exposure and the perpetuation of low socioeconomic status. Cliometrics Org. 2015. [Google Scholar]
- Neuwirth, L.S. Resurgent lead poisoning and renewed public attention towards environmental social justice issues: A review of current efforts and call to revitalize primary and secondary lead poisoning prevention for pregnant women, lactating mothers, and children within the US. Int. J. Occup. Environ. Health 2018, 24, 86–100. [Google Scholar] [PubMed]
- Katner, A.; Pieper, K.J.; Lambrinidou, Y.; Brown, K.; Hu, C.-Y.; Mielke, H.W.; Edwards, M.A. Weaknesses in federal drinking water regulations and public health policies that impede lead poisoning prevention and environmental justice. Environ. Justice 2016, 9, 109–117. [Google Scholar] [CrossRef]
- Sampson, R.J. Legacies of inequality, legacy lead exposures, and improving population well-being. Proc. Natl. Acad. Sci. USA 2022, 119, e2202401119. [Google Scholar] [CrossRef] [PubMed]
- Benfer, E.A. Contaminated childhood: How the United States failed to prevent the chronic lead poisoning of low-income children and communities of color. Harv. Envtl. L. Rev. 2017, 41, 493. [Google Scholar]
- Teye, S.O.; Yanosky, J.D.; Cuffee, Y.; Weng, X.; Luquis, R.; Farace, E.; Wang, L. Exploring persistent racial/ethnic disparities in lead exposure among American children aged 1–5 years: Results from NHANES 1999–2016. Int. Arch. Occup. Environ. Health 2021, 94, 723–730. [Google Scholar] [CrossRef] [PubMed]
- Benfer, E.A. Contaminated childhood: The chronic lead poisoning of low-income children and communities of color in the United States. Health Aff. Forefr. 2017. [Google Scholar] [CrossRef]
- Sorensen, L.C.; Fox, A.M.; Jung, H.; Martin, E.G. Lead exposure and academic achievement: Evidence from childhood lead poisoning prevention efforts. J. Popul. Econ. 2019, 32, 179–218. [Google Scholar] [CrossRef]
- Aizer, A.; Currie, J.; Simon, P.; Vivier, P. Lead Exposure and Racial Disparities in Test Scores. Brown Univ. Work. Pap 2015. [Google Scholar]
- Blackowicz, M.J.; Hryhorczuk, D.O.; Rankin, K.M.; Lewis, D.A.; Haider, D.; Lanphear, B.P.; Evens, A. The impact of low-level lead toxicity on school performance among Hispanic subgroups in the Chicago public schools. Int. J. Environ. Res. Public Health 2016, 13, 774. [Google Scholar] [CrossRef]
- Wodtke, G.T.; Ramaj, S.; Schachner, J. Toxic neighborhoods: The effects of concentrated poverty and environmental lead contamination on early childhood development. Demography 2022, 59, 1275–1298. [Google Scholar] [CrossRef]
- Winter, A.S.; Sampson, R.J. From lead exposure in early childhood to adolescent health: A Chicago birth cohort. Am. J. Public Health 2017, 107, 1496–1501. [Google Scholar] [CrossRef]
- Smith, P.P.; Nriagu, J.O. Lead poisoning and asthma among low-income and African American children in Saginaw, Michigan. Environ. Res. 2011, 111, 81–86. [Google Scholar] [CrossRef]
- Gellert, G.A.; Wagner, G.A.; Maxwell, R.M.; Moore, D.; Foster, L. Lead poisoning among low-income children in Orange County, California: A need for regionally differentiated policy. JAMA 1993, 270, 69–71. [Google Scholar] [CrossRef]
- Pickett, K.E.; Wilkinson, R.G. Income inequality and health: A causal review. Soc. Sci. Med. 2015, 128, 316–326. [Google Scholar] [CrossRef]
- Perron, T.; Hartt, K.; McCann, D.; McGowan, R.; Segers, N. Lead poisoning in populations with low-socio-economic status in the United States. Br. J. Sch. Nurs. 2018, 13, 332–336. [Google Scholar] [CrossRef]
- Jacobs, D.E. Environmental health disparities in housing. Am. J. Public Health 2011, 101, S115–S122. [Google Scholar] [CrossRef]
- Adamkiewicz, G.; Spengler, J.D.; Harley, A.E.; Stoddard, A.; Yang, M.; Alvarez-Reeves, M.; Sorensen, G. Environmental conditions in low-income urban housing: Clustering and associations with self-reported health. Am. J. Public Health 2014, 104, 1650–1656. [Google Scholar] [CrossRef] [PubMed]
- Yu, Y.; Tarpley, D.; Privette, J.L.; Goldberg, M.D.; Raja, M.R.V.; Vinnikov, K.Y.; Xu, H. Developing algorithm for operational GOES-R land surface temperature product. IEEE Trans. Geosci. Remote Sens. 2008, 47, 936–951. [Google Scholar]
- Yu, Y.; Yu, P. Land surface temperature product from the GOES-R series. In The GOES-R Series; Elsevier: Amsterdam, The Netherlands, 2020; pp. 133–144. [Google Scholar]
- Lee, J.; Dessler, A.E. Improved Surface Urban Heat Impact Assessment Using GOES Satellite Data: A Comparative Study With ERA-5. Geophys. Res. Lett. 2024, 51, e2023GL107364. [Google Scholar] [CrossRef]
- Lai, J.; Zhan, W.; Voogt, J.; Quan, J.; Huang, F.; Zhou, J.; Bechtel, B.; Hu, L.; Wang, K.; Cao, C. Meteorological controls on daily variations of nighttime surface urban heat islands. Remote Sens. Environ. 2021, 253, 112198. [Google Scholar] [CrossRef]
- Bechtel, B.; Demuzere, M.; Mills, G.; Zhan, W.; Sismanidis, P.; Small, C.; Voogt, J. SUHI analysis using Local Climate Zones—A comparison of 50 cities. Urban Clim. 2019, 28, 100451. [Google Scholar] [CrossRef]
- Lai, J.; Zhan, W.; Huang, F.; Voogt, J.; Bechtel, B.; Allen, M.; Peng, S.; Hong, F.; Liu, Y.; Du, P. Identification of typical diurnal patterns for clear-sky climatology of surface urban heat islands. Remote Sens. Environ. 2018, 217, 203–220. [Google Scholar] [CrossRef]
- Liu, Z.; Zhan, W.; Lai, J.; Bechtel, B.; Lee, X.; Hong, F.; Li, L.; Huang, F.; Li, J. Taxonomy of seasonal and diurnal clear-sky climatology of surface urban heat island dynamics across global cities. ISPRS J. Photogramm. Remote Sens. 2022, 187, 14–33. [Google Scholar] [CrossRef]
- Chang, Y.; Xiao, J.; Li, X.; Frolking, S.; Zhou, D.; Schneider, A.; Weng, Q.; Yu, P.; Wang, X.; Li, X. Exploring diurnal cycles of surface urban heat island intensity in Boston with land surface temperature data derived from GOES-R geostationary satellites. Sci. Total Environ. 2021, 763, 144224. [Google Scholar] [CrossRef] [PubMed]
- Zakšek, K.; Oštir, K. Downscaling land surface temperature for urban heat island diurnal cycle analysis. Remote Sens. Environ. 2012, 117, 114–124. [Google Scholar] [CrossRef]
- Zhou, J.; Chen, Y.; Zhang, X.; Zhan, W. Modelling the diurnal variations of urban heat islands with multi-source satellite data. Int. J. Remote Sens. 2013, 34, 7568–7588. [Google Scholar] [CrossRef]
- Stewart, I.; Krayenhoff, E.; Voogt, J.; Lachapelle, J.; Allen, M.; Broadbent, A. Time evolution of the surface urban heat island. Earth’s Future 2021, 9, e2021EF002178. [Google Scholar] [CrossRef]
- Hastie, T.J. Generalized additive models. In Statistical Models in S; Routledge: London, UK, 2017; pp. 249–307. [Google Scholar]
- Servén, D.; Brummitt, C. pyGAM Documentation. 2020. Available online: https://readthedocs.org/projects/pygam/downloads/pdf/latest/ (accessed on 15 January 2024).
- Virtanen, P.; Gommers, R.; Oliphant, T.E.; Haberland, M.; Reddy, T.; Cournapeau, D.; Burovski, E.; Peterson, P.; Weckesser, W.; Bright, J. SciPy 1.0: Fundamental algorithms for scientific computing in Python. Nat. Methods 2020, 17, 261–272. [Google Scholar] [CrossRef] [PubMed]
- Jordahl, K.; Van den Bossche, J.; Wasserman, J.; McBride, J.; Gerard, J.; Fleischmann, M.; Tratner, J.; Perry, M.; Farmer, C.; Hjelle, G.A. Geopandas/Geopandas: v0. 6.0; Zenodo: Genève, Switzerland, 2019. [Google Scholar]
- Tosi, S. Matplotlib for Python Developers; Packt Publishing Ltd.: Birmingham, UK, 2009. [Google Scholar]
- Markowitz, M.E.; Sinnett, M.; Rosen, J.F. A randomized trial of calcium supplementation for childhood lead poisoning. Pediatrics 2004, 113, e34–e39. [Google Scholar] [CrossRef]
- Bruening, K.; Kemp, F.W.; Simone, N.; Holding, Y.; Louria, D.B.; Bogden, J.D. Dietary calcium intakes of urban children at risk of lead poisoning. Environ. Health Perspect. 1999, 107, 431–435. [Google Scholar] [CrossRef]
- Flora, S.J.; Flora, G.; Saxena, G. Environmental occurrence, health effects and management of lead poisoning. In Lead; Elsevier: Amsterdam, The Netherlands, 2006; pp. 158–228. [Google Scholar]
- Gascon, F.; Bouzinac, C.; Thépaut, O.; Jung, M.; Francesconi, B.; Louis, J.; Lonjou, V.; Lafrance, B.; Massera, S.; Gaudel-Vacaresse, A. Copernicus Sentinel-2A calibration and products validation status. Remote Sens. 2017, 9, 584. [Google Scholar] [CrossRef]
- Son, J.-Y.; Liu, J.C.; Bell, M.L. Temperature-related mortality: A systematic review and investigation of effect modifiers. Environ. Res. Lett. 2019, 14, 073004. [Google Scholar] [CrossRef]
- Lee, J.; Dessler, A.E. Future Temperature-Related Deaths in the US: The Impact of Climate Change, Demographics, and Adaptation. GeoHealth 2023, 7, e2023GH000799. [Google Scholar] [CrossRef]
- Hajat, S.; Vardoulakis, S.; Heaviside, C.; Eggen, B. Climate change effects on human health: Projections of temperature-related mortality for the UK during the 2020s, 2050s and 2080s. J. Epidemiol. Community Health 2014, 68, 641–648. [Google Scholar] [CrossRef]
- Vonesch, N.; D’Ovidio, M.C.; Melis, P.; Remoli, M.E.; Grazia Ciufolini, M.; Tomao, P. Climate change, vector-borne diseases and working population. Ann. Dell’istituto Super. Sanita 2016, 52, 397–405. [Google Scholar]
- Githeko, A.K.; Lindsay, S.W.; Confalonieri, U.E.; Patz, J.A. Climate change and vector-borne diseases: A regional analysis. Bull. World Health Organ. 2000, 78, 1136–1147. [Google Scholar] [PubMed]
- Semenza, J.C.; Suk, J.E. Vector-borne diseases and climate change: A European perspective. FEMS Microbiol. Lett. 2018, 365, fnx244. [Google Scholar] [CrossRef]
- Brisbois, B.W.; Ali, S.H. Climate change, vector-borne disease and interdisciplinary research: Social science perspectives on an environment and health controversy. EcoHealth 2010, 7, 425–438. [Google Scholar] [CrossRef]
- Palinkas, L.A.; Wong, M. Global climate change and mental health. Curr. Opin. Psychol. 2020, 32, 12–16. [Google Scholar] [CrossRef]
- Berry, H.L.; Bowen, K.; Kjellstrom, T. Climate change and mental health: A causal pathways framework. Int. J. Public Health 2010, 55, 123–132. [Google Scholar] [CrossRef]
- Charlson, F.; Ali, S.; Benmarhnia, T.; Pearl, M.; Massazza, A.; Augustinavicius, J.; Scott, J.G. Climate change and mental health: A scoping review. Int. J. Environ. Res. Public Health 2021, 18, 4486. [Google Scholar] [CrossRef]
- Trombley, J.; Chalupka, S.; Anderko, L. Climate change and mental health. AJN Am. J. Nurs. 2017, 117, 44–52. [Google Scholar] [CrossRef]
- D’Amato, G.; Cecchi, L.; D’Amato, M.; Annesi-Maesano, I. Climate change and respiratory diseases. Eur. Respir. Rev. 2014, 23, 161–169. [Google Scholar] [CrossRef]
- Barnes, C.S. Impact of climate change on pollen and respiratory disease. Curr. Allergy Asthma Rep. 2018, 18, 59. [Google Scholar] [CrossRef]
- Ayres, J.; Forsberg, B.; Annesi-Maesano, I.; Dey, R.; Ebi, K.; Helms, P.; Medina-Ramon, M.; Windt, M.; Forastiere, F. Climate change and respiratory disease: European Respiratory Society position statement. Eur. Respir. J. 2009, 34, 295–302. [Google Scholar] [CrossRef] [PubMed]
- Demain, J.G. Climate change and the impact on respiratory and allergic disease: 2018. Curr. Allergy Asthma Rep. 2018, 18, 22. [Google Scholar] [CrossRef] [PubMed]
- Shrestha, J.; Khan, R.K.; McClintock, S.; DeGroote, J.; Zeman, C.L. Correlations between Educational Struggle, Toxic Sites by School District and Demographic Variables, with Geographical Information System Projections. Int. J. Environ. Res. Public Health 2023, 20, 7160. [Google Scholar] [CrossRef] [PubMed]
Figure 1.
Map of percentage of children with over 5 µg/dL of BLLs (LP) in Chicago area for 2019–2021 period, aggregated at the zip code level.
Figure 1.
Map of percentage of children with over 5 µg/dL of BLLs (LP) in Chicago area for 2019–2021 period, aggregated at the zip code level.
Figure 2.
Zip-code-level map of socioeconomic variables and land surface temperature metrics. (a) Average per capita income (income), (b) unemployment rate (unemployment), (c) percentage of population with a high school diploma (education), (d) years after the building was built (building age), (e) average daytime (10:00~15:00) LST anomaly for summertime (June, July, and August) from 2018 to 2021, and (f) average nighttime (00:00~05:00) LST anomaly for summertime from 2018 to 2021.
Figure 2.
Zip-code-level map of socioeconomic variables and land surface temperature metrics. (a) Average per capita income (income), (b) unemployment rate (unemployment), (c) percentage of population with a high school diploma (education), (d) years after the building was built (building age), (e) average daytime (10:00~15:00) LST anomaly for summertime (June, July, and August) from 2018 to 2021, and (f) average nighttime (00:00~05:00) LST anomaly for summertime from 2018 to 2021.
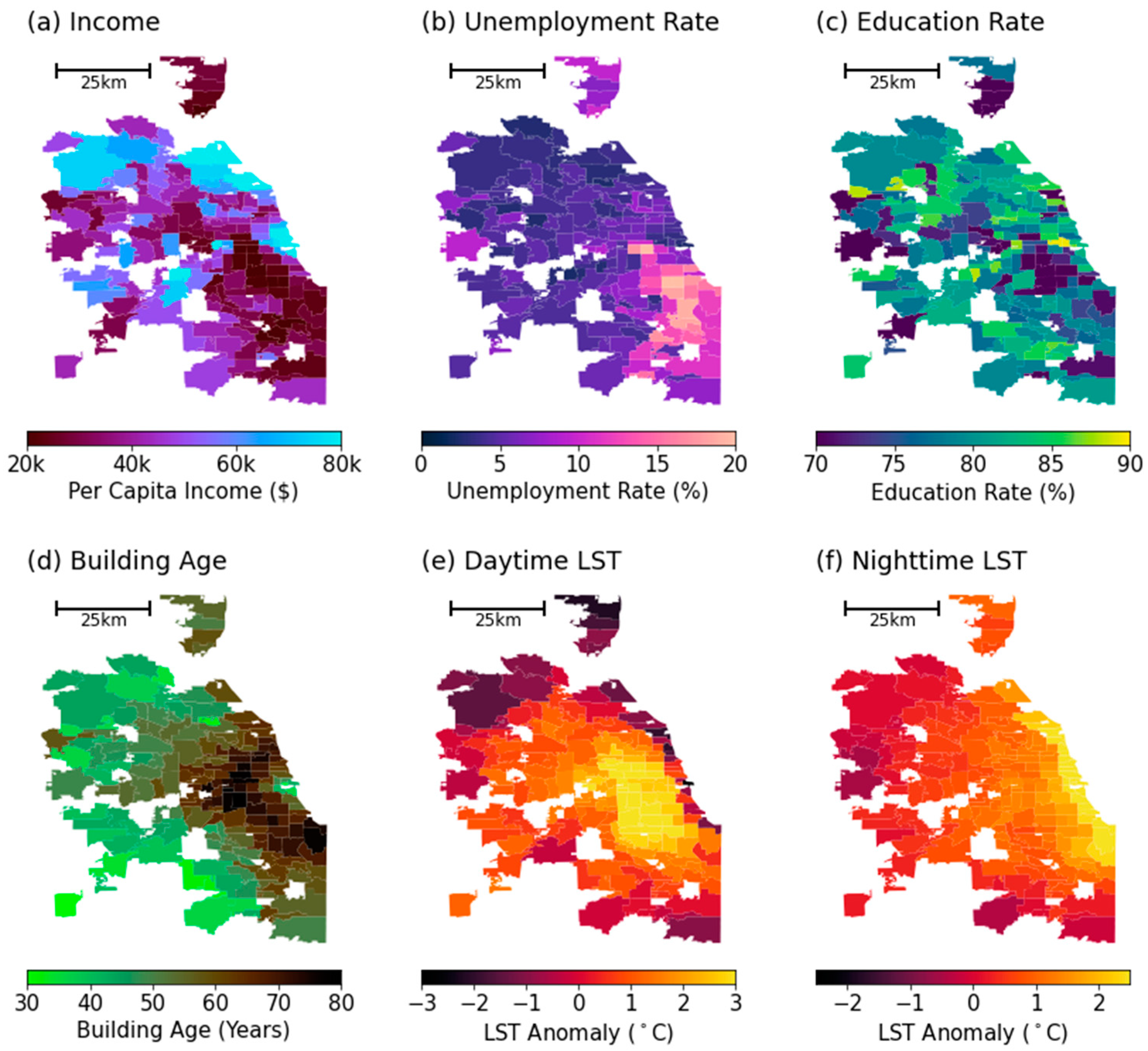
Figure 3.
Heatmap displaying the cross-correlation matrix of predictor variables with correlation coefficients.
Figure 3.
Heatmap displaying the cross-correlation matrix of predictor variables with correlation coefficients.
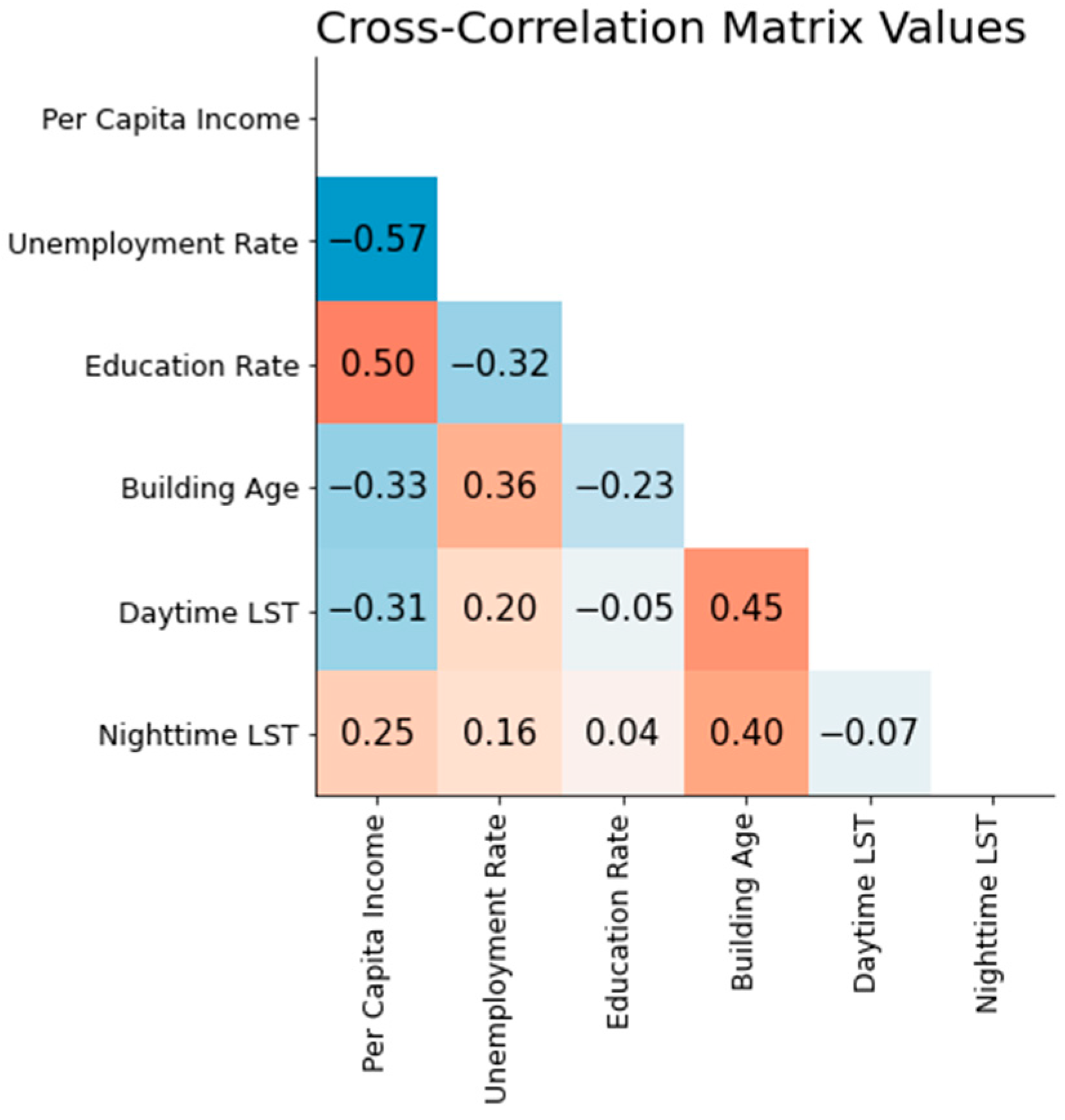
Figure 4.
Comparative distribution of predictor variables between Q1 (left) and Q4 (right) LP rate percentile groups. The variables include (a) per capita income, (b) unemployment rate, (c) education rate, (d) building age, (e) daytime LST, and (f) nighttime LST. Within each box plot, the median values are indicated by red lines, while the boxes represent the interquartile range (IQR), spanning from the 25th to the 75th percentile. The whiskers extend to 1.5 times the IQR.
Figure 4.
Comparative distribution of predictor variables between Q1 (left) and Q4 (right) LP rate percentile groups. The variables include (a) per capita income, (b) unemployment rate, (c) education rate, (d) building age, (e) daytime LST, and (f) nighttime LST. Within each box plot, the median values are indicated by red lines, while the boxes represent the interquartile range (IQR), spanning from the 25th to the 75th percentile. The whiskers extend to 1.5 times the IQR.
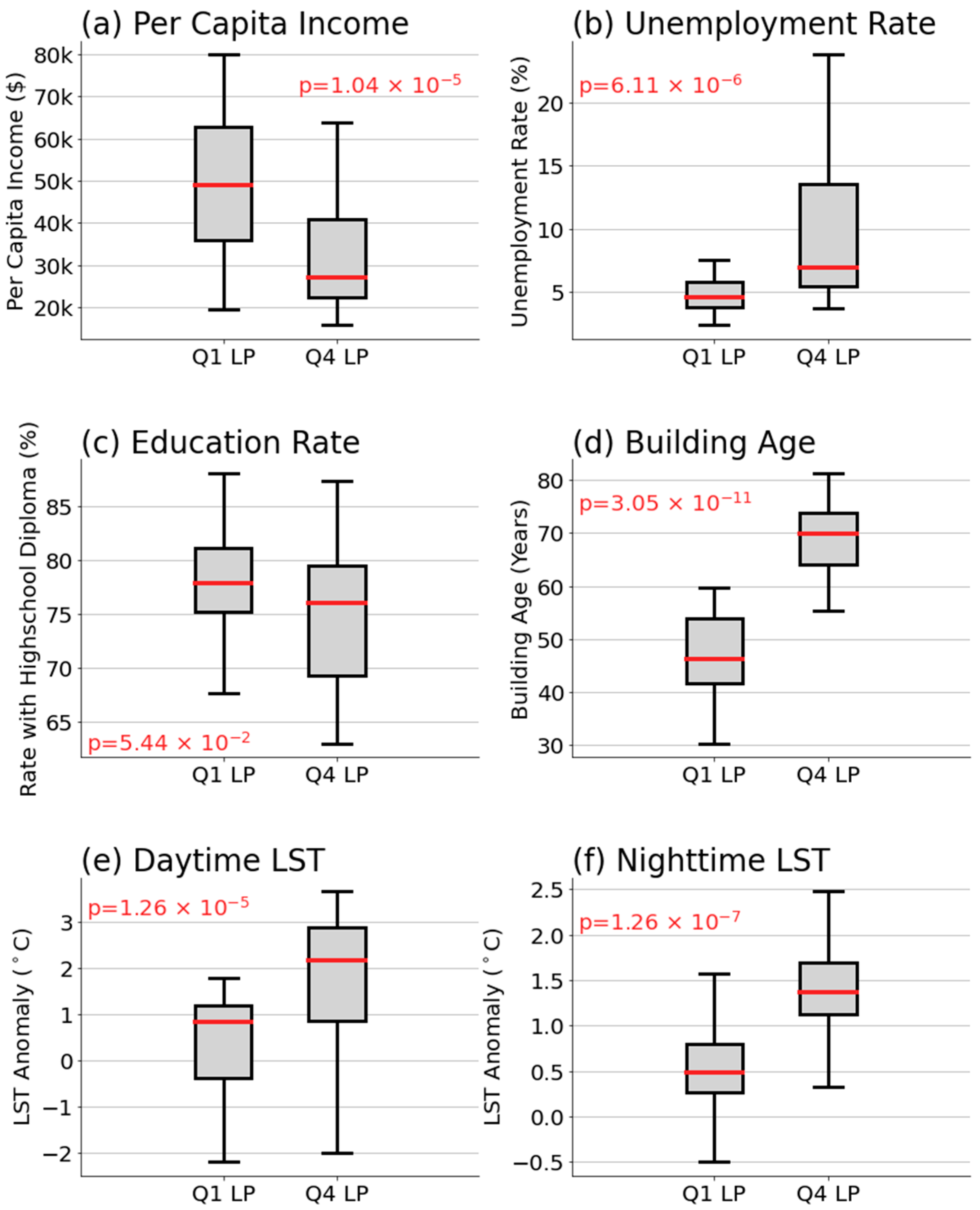
Figure 5.
Partial dependence of each predictor variable, derived from GAM regression. The variables include (a) per capita income, (b) unemployment rate, (c) education rate, (d) building age, (e) daytime LST, and (f) nighttime LST. The black line represents the estimated partial dependence, while the gray shaded area shows the 90% confidence interval of the dependence. Blue, orange, and green dots represent the median values for each racial group, while the corresponding error bars denote the 5–95th percentile interval of predictor variables, for each racial group.
Figure 5.
Partial dependence of each predictor variable, derived from GAM regression. The variables include (a) per capita income, (b) unemployment rate, (c) education rate, (d) building age, (e) daytime LST, and (f) nighttime LST. The black line represents the estimated partial dependence, while the gray shaded area shows the 90% confidence interval of the dependence. Blue, orange, and green dots represent the median values for each racial group, while the corresponding error bars denote the 5–95th percentile interval of predictor variables, for each racial group.
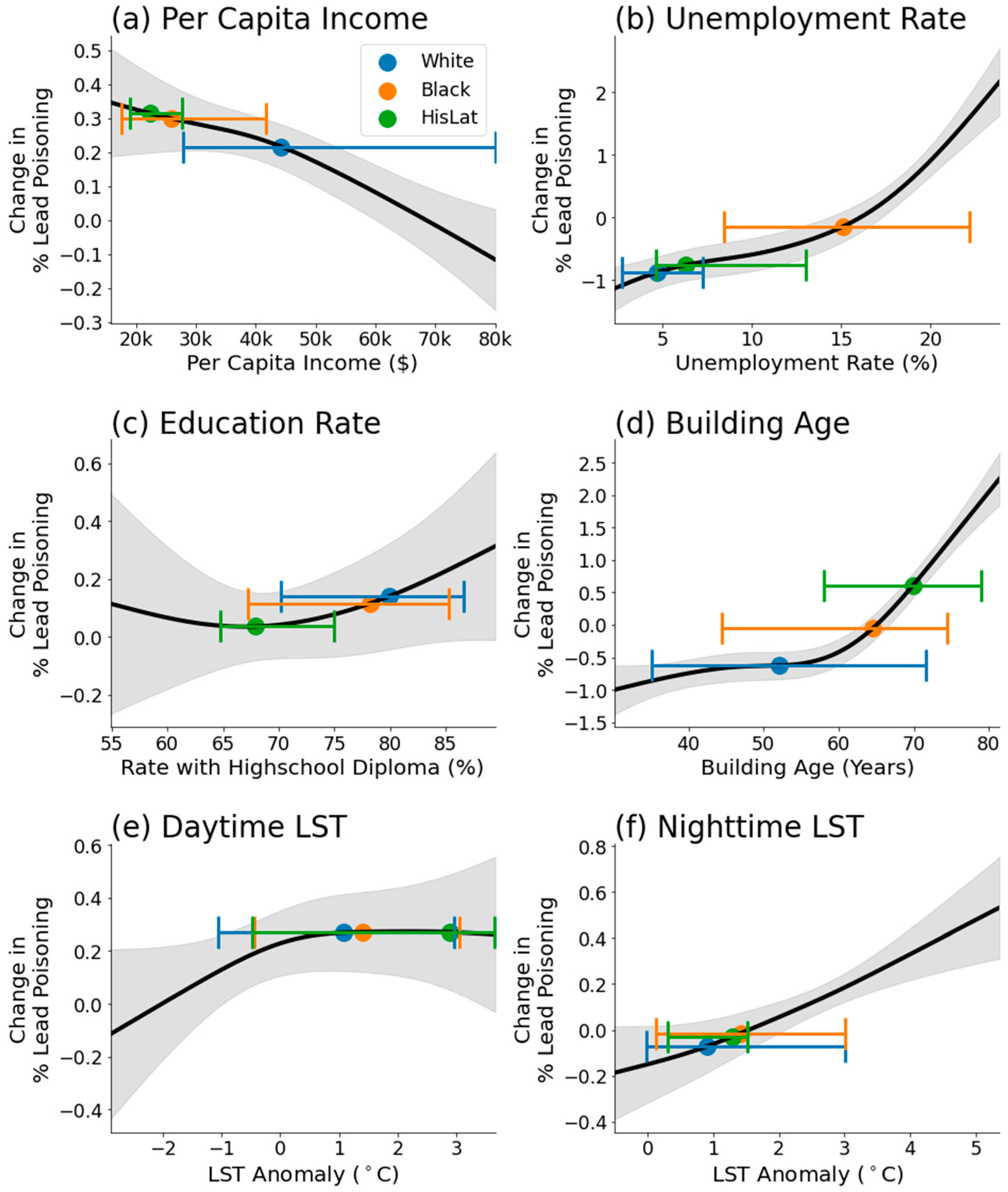
Figure 6.
Bar graph showing the relative change in LP rates for Black and Hispanic/Latino (HisLat) populations in comparison to the White population. Each bar is segmented to demonstrate the contribution of different predictor variables: per capita income (blue), unemployment rate (orange), building age (green), daytime LST (purple), and nighttime LST (red).
Figure 6.
Bar graph showing the relative change in LP rates for Black and Hispanic/Latino (HisLat) populations in comparison to the White population. Each bar is segmented to demonstrate the contribution of different predictor variables: per capita income (blue), unemployment rate (orange), building age (green), daytime LST (purple), and nighttime LST (red).
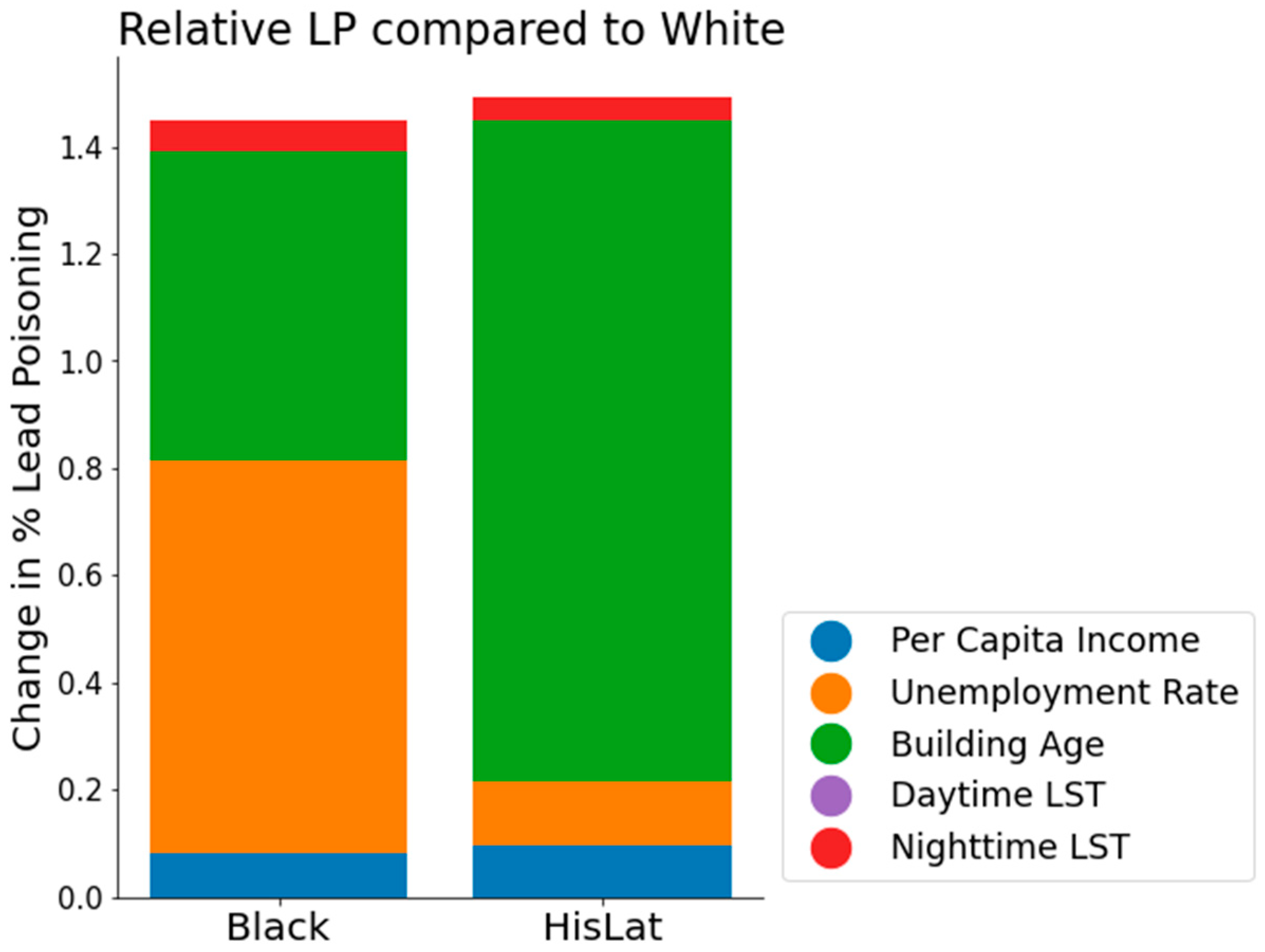
Disclaimer/Publisher’s Note: The statements, opinions and data contained in all publications are solely those of the individual author(s) and contributor(s) and not of MDPI and/or the editor(s). MDPI and/or the editor(s) disclaim responsibility for any injury to people or property resulting from any ideas, methods, instructions or products referred to in the content. |
[ad_2]