Generalised Linear Modelling for Construction Waste Estimation in Residential Projects: Case Study in New Zealand
[ad_1]
1. Introduction
2. Construction Waste Prediction and Quantification
3. Construction Waste Generation in Residential Projects in New Zealand
4. Methodology
To accomplish the aforementioned research objective of developing a model for estimating construction waste during the early design stage of residential construction projects, a quantitative approach was employed in this study. A total of 213 detached residential projects were utilised, and both waste quantities and building designs were taken into consideration. These projects were systematically selected to represent the typical range of construction styles and sizes observed in New Zealand, encompassing the work of several small builders. The sample spanned a period of approximately seven years, from 2015 to 2023. Within the sample, there were residential projects completed by a national builder (n = 162) as well as several small builders located in Palmerston North and Manawatu (n = 51) in New Zealand. A smaller subset of the sample comprised architectural-style homes. The selected projects predominantly featured detached houses with raft slab foundations, pre-nail timber frames, colour steel roofing, and board/sheet and brick or stone cladding. The data collected for analysis were divided into response variables and predictor variables.
4.1. Response Variable
4.2. Predictor Variables
5. Results
5.1. Predicting Waste Volume without Roof Parameters
5.2. Predicting Waste Volume with Roof Parameters
5.3. Prediction Tool
5.4. Predicting Waste Volume without Roof Parameters
5.5. Predicting Waste Volume with Roof Parameters
5.6. Prediction Tool
6. Discussion
This article presents a comprehensive investigation into the residential building design factors influencing construction waste volume, utilising multiple predictive models. The study primarily focuses on identifying the best-fit model to enhance the accuracy of waste volume predictions. As the Pearson correlation analysis shows a strong correlation between floor area and roof parameters, the analysis involves three distinct models (model 2, model 3, and model 4), each incorporating different sets of predictor variables. The findings reveal intriguing insights into the correlation between various construction parameters and waste generation.
The increasing importance of sustainable construction practices has spurred research into understanding and predicting construction waste volume. This study delves into three predictive models, aiming to identify the most influential factors and enhance prediction accuracy. Notably, the research explores the impact of roof parameters on waste generation, shedding light on previously underexplored dimensions of construction waste modelling.
The initial model (model 1) establishes a strong correlation between construction waste volume and fundamental factors such as working days, corners, and floor area. Intriguingly, residential projects led by small builders exhibit significantly higher waste generation, providing valuable insights for waste management strategies. Model 2 introduces enhanced accuracy (80.00%) by incorporating additional predictor variables, notably the presence of multiple bathrooms. Despite the increased accuracy, the study suggests that the fitness of model 2 is not significantly different from model 1, emphasising the practicality of model 2 for prediction without roof parameters. The incorporation of PCA elucidates the dominant factors contributing to waste generation. PC1, representing overall built area, emerges as a significant contributor to waste volume. PC2, influenced by internal wall length and roof area, provides supplementary insights. The combination of PC1 and PC2 explains a substantial 97.34% of the total variance, underscoring their importance in waste volume prediction. Model 3 introduces roof parameters, showing a lower AIC (71.48) and an accuracy of 75.00%. The negative correlation observed between PC1, PC2, and waste volume suggests that reducing the built area may mitigate waste generation. Moreover, the study identifies a positive correlation between adding extra levels to a structure and increased waste volume. The final model (model 4) incorporates roof cladding type, working days, and bathrooms, achieving a remarkable 100.00% accuracy in test data predictions. The positive correlation between PC1 and waste volume is reaffirmed. Importantly, model 4 does not exhibit a significant difference in fitness compared to model 3, signifying its applicability for accurate predictions with roof parameters.
7. Conclusions
This study employed generalised linear models to examine the relationship between design features and waste generation in residential buildings within New Zealand. Generalised linear models were chosen due to their ability to offer a more flexible approximation of the distribution of the response variable, surpassing the capabilities of multiple regression models. Consequently, this approach enabled the development of a model with a high level of accuracy, estimating construction waste generation in residential projects within New Zealand at approximately 80% accuracy.
The analysis revealed that small builders tend to generate a greater amount of waste in residential projects compared to national builders, as supported by compelling evidence obtained from the model. Furthermore, the results indicate that enhancing the accuracy of waste estimation can be achieved by incorporating additional design features into the model. Principal component analysis demonstrated that certain design features, specifically overall built area, internal wall length, and roof area, significantly contributed to the variance in waste volumes. This implies that these particular design aspects exert a substantial influence on waste generation, surpassing the impact of other design features. Additionally, the findings indicate that the overall waste volume can be reduced by minimising the number of bathrooms and the overall built area. The implications of this study’s findings are particularly valuable to construction stakeholders, especially those involved in the design phase, as they offer insights into minimising waste generation by optimising design parameters. By implementing the recommendations derived from this study, construction industry professionals can contribute to sustainable practices and waste reduction in residential projects.
Author Contributions
Conceptualisation, N.D. and R.K.; methodology, N.D., G.W. and H.M.E.; formal analysis, H.M.E. and R.K.; investigation, N.D., H.M.E. and R.K.; resources, N.D.; data curation, N.D., R.K. and H.M.E.; writing—original draft preparation, N.D., H.M.E. and G.W.; writing—review and editing, N.D.; visualisation, N.D., H.M.E. and R.K.; supervision, N.D.; project administration, N.D.; funding acquisition, N.D. All authors have read and agreed to the published version of the manuscript.
Funding
This research was funded by Auckland Council Waste Management and Innovation Fund, grant number WMIF2002-026.
Institutional Review Board Statement
The research project was conducted according to the guidelines of Massey University Human Ethics Committees. The project has been evaluated by peer review and judged to be low risk. Human Ethics Notification–4000024389.
Data Availability Statement
Data available upon request.
Conflicts of Interest
The authors declare no conflict of interest.
References
- Tafesse, S.; Girma, Y.E.; Dessalegn, E. Analysis of the socio-economic and environmental impacts of construction waste and management practices. Heliyon 2022, 8, e09169. [Google Scholar] [CrossRef]
- Skoyles, E.R. Waste Prevention on Site; BT Batsford Limited.: London, UK, 1987. [Google Scholar]
- European Directive 2008/98/EC; Waste Framework Directive Targeting Construction and Demolition Waste and Waste from Households. European Council: Brussels, Belgium, 2008.
- Domingo, N.; Batty, T. Construction waste modelling for residential construction projects in New Zealand to enhance design outcomes. Waste Manag. 2021, 120, 484–493. [Google Scholar] [CrossRef]
- Mah, C.M.; Fujiwara, T.; Ho, C.S. Environmental impacts of construction and demolition waste management alternatives. Chem. Eng. Trans. 2018, 63, 343–348. [Google Scholar]
- Bajjou, M.S.; Chafi, A. Exploring the critical waste factors affecting construction projects. Eng. Constr. Arch. Manag. 2021, 29, 2268–2299. [Google Scholar] [CrossRef]
- Llatas, C.; Osmani, M. Development and validation of a building design waste reduction model. Waste Manag. 2016, 56, 318–336. [Google Scholar] [CrossRef] [PubMed]
- Luangcharoenrat, C.; Intrachooto, S.; Peansupap, V.; Sutthinarakorn, W. Factors Influencing Construction Waste Generation in Building Construction: Thailand’s Perspective. Sustainability 2019, 11, 3638. [Google Scholar] [CrossRef]
- Udawatta, N.; Zuo, J.; Chiveralla, K.; Zillante, G. Improving waste management in construction projects: An Australian study. Resour. Conserv. Recycl. 2015, 101, 73–83. [Google Scholar] [CrossRef]
- Esa, M.R.; Halog, A.; Rigamonti, L. Strategies for minimizing construction and demolition wastes in Malaysia. Resour. Conserv. Recycl. 2017, 120, 219–229. [Google Scholar] [CrossRef]
- Parisi Kern, A.; Dias, M.; Kulakowski, M.P.; Gomas, L.P. Waste generated in high rise buildings construction: A quantification model based on statistical multiple regression. Waste Manag. 2015, 39, 35–44. [Google Scholar] [CrossRef]
- Osmani, M.; Glass, J.; Price, A. Architects’ perspectives on construction waste reduction by design. Waste Manag. 2008, 28, 1147–1158. [Google Scholar] [CrossRef]
- Ripley, B.; Venables, B.; Bates, D.M.; Hornik, K.; Gebhardt, A.; Firth, D.; Ripley, M.B. Package ‘mass’. Cran. R 2013, 538, 113–120. [Google Scholar]
- Bakshan, A.; Srour, I.; Chehab, G.; El-Fadel, M. A field based methodology for estimating waste generation rates at various stages of construction projects. Resour. Conserv. Recycl. 2015, 100, 70–80. [Google Scholar] [CrossRef]
- Li, J.; Ding, Z.; Mi, X.; Wang, J. A model for estimating construction waste generation index for building project in China. Resour. Conserv. Recycl. 2013, 74, 20–26. [Google Scholar] [CrossRef]
- Islam, R.; Nazifa, T.H.; Yuniarto, A.; Uddin, A.S.; Salmiati, S.; Shahid, S. An empirical study of construction and demolition waste generation and implication of recycling. Waste Manag. 2019, 95, 10–21. [Google Scholar] [CrossRef] [PubMed]
- Sáez, P.V.; Porras-Amores, C.; del Río Merino, M. New quantification proposal for construction waste generation in new residential constructions. J. Clean. Prod. 2015, 102, 58–65. [Google Scholar] [CrossRef]
- Maués, L.M.F.; Nascimento, B.D.M.O.D.; Lu, W.; Xue, F. Estimating construction waste generation in residential buildings: A fuzzy set theory approach in the Brazilian Amazon. J. Clean. Prod. 2020, 265, 121779. [Google Scholar] [CrossRef]
- Hu, R.; Chen, K.; Chen, W.; Wang, Q.; Luo, H. Estimation of construction waste generation based on an improved on-site measurement and SVM-based prediction model: A case of commercial buildings in China. Waste Manag. 2021, 126, 791–799. [Google Scholar] [CrossRef] [PubMed]
- Lee, D.; Kim, S.; Kim, S. Development of Hybrid Model for Estimating Construction Waste for Multifamily Residential Buildings Using Artificial Neural Networks and Ant Colony Optimization. Sustainability 2016, 8, 870. [Google Scholar] [CrossRef]
- Liu, J.; Chen, J.; Tang, K. A Method for Estimation of the On-Site Construction Waste Quantity of Residential Projects. In Proceedings of the ICCREM 2018, Charleston, SC, USA, 9–10 August 2018; pp. 225–231. [Google Scholar] [CrossRef]
- Bakchan, A.; Faust, K.M.; Leite, F. Seven-dimensional automated construction waste quantification and management framework: Integration with project and site planning. Resour. Conserv. Recycl. 2019, 146, 462–474. [Google Scholar] [CrossRef]
- Guerra, B.C.; Bakchan, A.; Leite, F.; Faust, K.M. BIM-based automated construction waste estimation algorithms: The case of concrete and drywall waste streams. Waste Manag. 2019, 87, 825–832. [Google Scholar] [CrossRef]
- Mercader-Moyano, P.; Ramírez-De-Arellano-Agudo, A. Selective classification and quantification model of C&D waste from material resources consumed in residential building construction. Waste Manag. Res. 2013, 31, 458–474. [Google Scholar] [CrossRef] [PubMed]
- Ghose, A.; Pizzol, M.; McLaren, S.J. Consequential LCA modelling of building refurbishment in New Zealand- an evaluation of resource and waste management scenarios. J. Clean. Prod. 2017, 165, 119–133. [Google Scholar] [CrossRef]
- Stats NZ. 2024. Available online: https://www.stats.govt.nz/ (accessed on 20 February 2024).
- MBIE. Building and Construction Sector Trends Biannual Snapshot: May 2022. Available online: https://www.mbie.govt.nz/building-and-energy/building/building-system-insights-programme/sector-trends-reporting/biannual-snapshots/november-2022/#:~:text=The%20sector%20had%207%2C035%20more,same%20time%20period%20in%202021 (accessed on 31 January 2024).
- MfE.2023. Waste Generation and Disposal in New Zealand. Available online: https://environment.govt.nz/facts-and-science/waste/waste-facilities-and-disposal/#about-the-data (accessed on 30 September 2023).
- Sáez, P.V.; del Río Merino, M.; Porras-Amores, C.; González, A.S.-A. European Legislation and Implementation Measures in the Management of Construction and Demolition Waste. Open Constr. Build. Technol. J. 2011, 5, 56–161. [Google Scholar]
- Mahayuddin, S.; Pereira, J.J.; Badaruzzaman, W.H.W.; Mokhtar, M.B. Construction Waste Index for Waste Control in Residential House Project. In Proceedings of the SB 10 New Zealand, Te Papa, New Zealand, 26–28 May 2010. [Google Scholar]
- Sáez, P.V.; Merino, M.d.R.; Porras-Amores, C.; González, A.S.-A. Assessing the accumulation of construction waste generation during residential building construction works. Resour. Conserv. Recycl. 2014, 93, 67–74. [Google Scholar] [CrossRef]
- Poon, C.-S.; Yu, A.T.; Jaillon, L. Reducing building waste at construction sites in Hong Kong. Constr. Manag. Econ. 2004, 22, 461–470. [Google Scholar] [CrossRef]
- Nagalli, A. Estimation of construction waste generation using machine learning. Proc. Inst. Civ. Eng.-Waste Resour. Manag. 2021, 174, 22–31. [Google Scholar] [CrossRef]
- Tuszynski, J.; Khachatryan, M.H. Package ‘caTools’. Available online: https://cran.r-project.org/web/packages/caTools/caTools.pdf (accessed on 30 September 2023).
- R Core Team. R: A Language and Environment for Statistical Computing; R Foundation for Statistical Computing: Vienna, Austria, 2022; Available online: http://www.R-project.org (accessed on 31 October 2023).
- Marschner, I.; Donoghoe, M.W.; Donoghoe, M.M.W. Package ‘glm2’. 2018. Available online: https://CRAN.R-project.org/package=glm2 (accessed on 20 February 2024).
- Qiao, L.; Liu, D.; Yuan, X.; Wang, Q.; Ma, Q. Generation and Prediction of Construction and Demolition Waste Using Exponential Smoothing Method: A Case Study of Shandong Province, China. Sustainability 2020, 12, 5094. [Google Scholar] [CrossRef]
- Ripley, B. Choose a Model by AIC in a Stepwise Algorithm; R Doc: Vienna, Austria, 2015. [Google Scholar]
- Fox, J.; Weisberg, S.; Adler, D.; Bates, D.; Baud-Bovy, G.; Ellison, S.; Graves, S. Package ‘car’; R Foundation for Statistical Computing: Vienna, Austria, 2012; p. 16. [Google Scholar]
- Liu, H.; Sydora, C.; Altaf, M.S.; Han, S.; Al-Hussein, M. Towards sustainable construction: BIM-enabled design and planning of roof sheathing installation for prefabricated buildings. J. Clean. Prod. 2019, 235, 1189–1201. [Google Scholar] [CrossRef]
- Kotu, V.; Deshpande, B. Chapter 2—Data Science Process. In Data Science, 2nd ed.; Kotu, V., Deshpande, B., Eds.; Morgan Kaufmann: Burlington, MA, USA, 2019; pp. 19–37. [Google Scholar]
- Dobson, A.J.; Barnett, A.G. An Introduction to Generalized Linear Models, 4th ed.; Chapman and Hall/CRC: Boca Raton, FL, USA, 2018. [Google Scholar] [CrossRef]
Figure 1.
Construction waste estimation methods.
Figure 1.
Construction waste estimation methods.
Figure 2.
Waste volume from construction of residential buildings.
Figure 2.
Waste volume from construction of residential buildings.
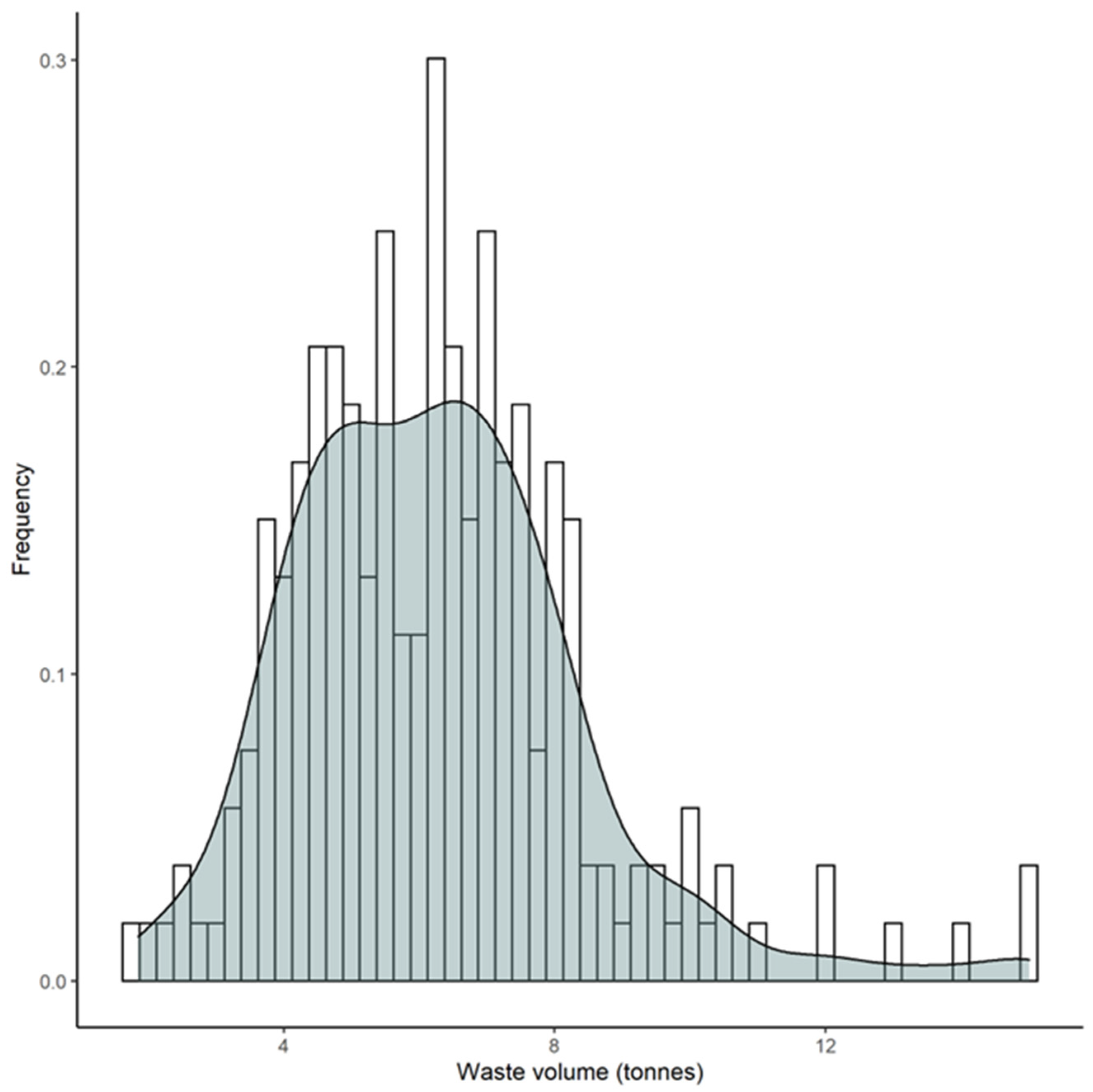
Figure 3.
Correlation analysis of variables.
Figure 3.
Correlation analysis of variables.
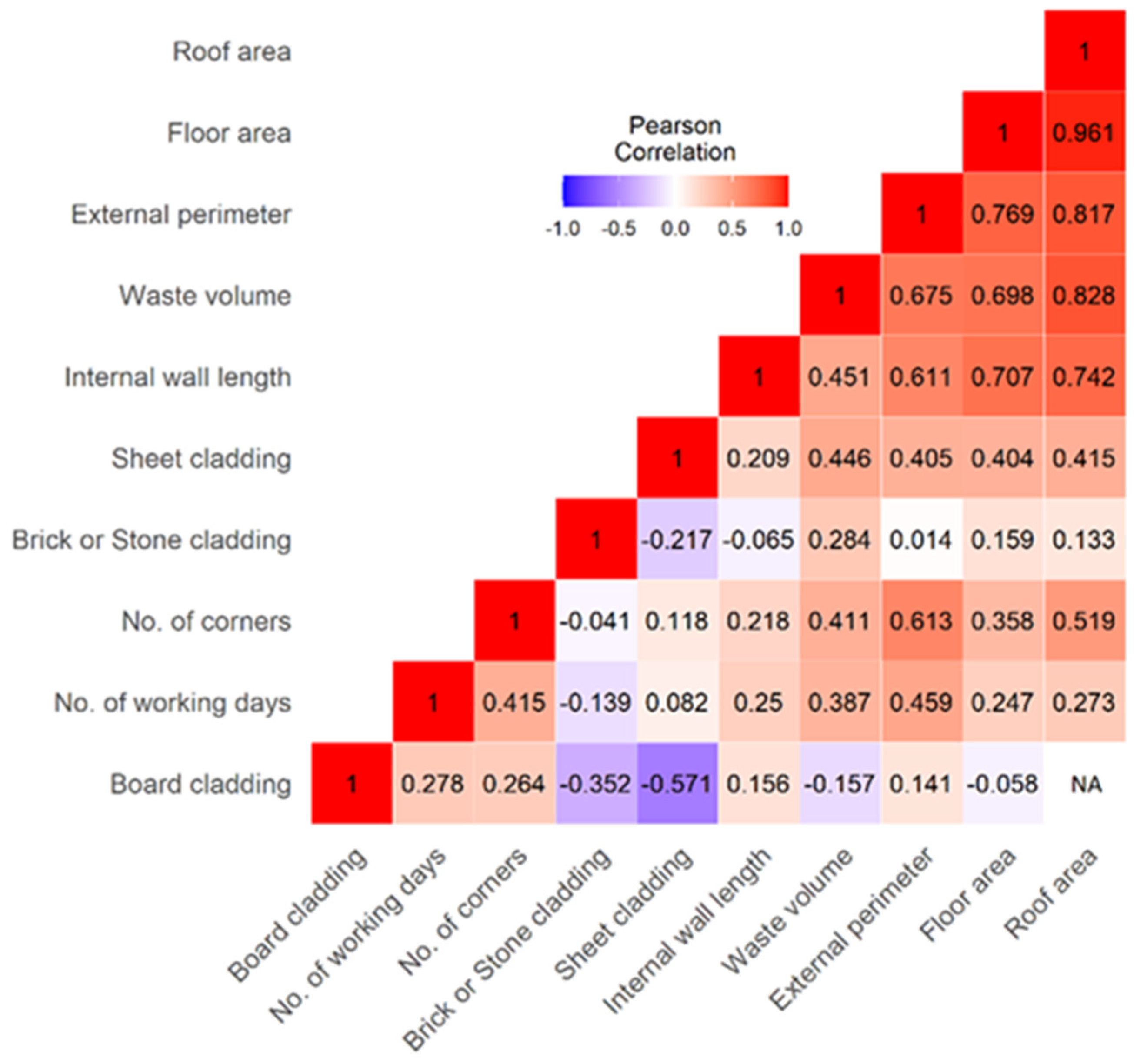
Table 1.
Summary of numerical variables.
Table 1.
Summary of numerical variables.
Variables | n | Mean ± SD | Median | Minimum | Maximum | Skewness | Kurtosis |
---|---|---|---|---|---|---|---|
Response Variable | |||||||
Waste volume (tonnes) | 213 | 6.28 ± 2.16 | 6.20 | 1.85 | 15.00 | 1.08 | 2.40 |
Predictor Variables | |||||||
Working days (days) | 213 | 139.02 ± 33.97 | 132.00 | 67.00 | 310.00 | 1.36 | 3.11 |
Floor area (m2) | 213 | 192.38 ± 41.45 | 192.00 | 89.60 | 337.90 | 0.55 | 1.13 |
External perimeter (m) | 213 | 72.95 ± 17.32 | 69.30 | 40.00 | 154.00 | 1.75 | 4.51 |
Internal wall length (m) | 213 | 67.51 ± 19.88 | 63.00 | 13.00 | 165.00 | 1.19 | 3.49 |
Corners (count) | 213 | 18.70 ± 6.50 | 17.00 | 9.00 | 46.00 | 1.43 | 2.30 |
Board cladding (m2) | 213 | 50.92 ± 56.16 | 34.00 | 0.00 | 224.00 | 0.84 | −0.30 |
Sheet cladding (m2) | 213 | 85.92 ± 75.38 | 77.00 | 0.00 | 384.00 | 0.70 | 0.35 |
Brick or stone (m2) | 213 | 26.83 ± 55.81 | 0.00 | 0.00 | 240.00 | 2.00 | 2.83 |
Roof area (m2) | 51 | 299.76 ± 51.26 | 291.00 | 211.00 | 460.00 | 0.94 | 1.51 |
Table 2.
Summary of categorical variables.
Table 2.
Summary of categorical variables.
Category | Levels |
---|---|
House type | Single-family houses (n = 169) |
Houses with a self-contained flat (n = 44) * | |
Number of stories | 1 (n = 188) |
1.5 (n = 1) | |
2 (n = 24) | |
Number of bathrooms | 1 (n = 6) |
1.5 (n = 1) | |
2 (n = 101) | |
2.5 (n = 39) | |
3 (n = 54) | |
3.5 (n = 7) | |
4 (n = 4) | |
5 (n = 1) | |
Roof cladding type (for small builders only) | Pressed metal (n = 12) |
Steel (n = 39) | |
Builder type | National builder (n = 162) |
Small builders (n = 51) |
Table 3.
Best-fitting generalised linear model (model 1) for residential buildings.
Table 3.
Best-fitting generalised linear model (model 1) for residential buildings.
Estimate | Std. Error | t Value | p Value | |
---|---|---|---|---|
(Intercept) | 0.352 | 0.094 | 3.754 | 0.000 |
Working days | 0.004 | 0.001 | 6.858 | 0.000 |
Floor area | 0.003 | 0.000 | 6.868 | 0.000 |
Number of corners | 0.008 | 0.003 | 2.905 | 0.004 |
Builder type—small builders | 0.372 | 0.046 | 8.090 | 0.000 |
Table 4.
Selected list of models with more than 56% accuracy.
Table 4.
Selected list of models with more than 56% accuracy.
Model Name | AIC | Accuracy of Prediction |
---|---|---|
Model 1: volume ~ working days + floor area + corners + type of builder | 516.28 | 56.00% |
Model 2: volume ~ working days + floor area + internal wall length + external perimeter + board cladding + sheet cladding + brick or stone + stories + bathrooms + house type + corners + type of builder | 528.25 | 80% |
Model 3: volume ~ stories + sheet cladding + PC1 + PC2 | 71.48 | 75.00% |
Model 4: volume ~ working days + PC1 + PC2 + roof clad + sheet cladding + bathrooms + stories | 82.40 | 100.00% |
Model 5: volume ~ working days + floor area + bathrooms + corners + type of builder | 72.00% | |
Model 6: volume ~ working days + floor area + bathrooms + corners + type of builder + stories | 72.00% | |
Model 7: volume ~ working days + floor area + bathrooms + corners + type of builder + house type | 72.00% | |
Model 8: volume ~ working days + floor area + bathrooms + corners + type of builder + sheet cladding | 72.00% | |
Model 9: volume ~ working days + floor area + bathrooms + corners + type of builder + sheet cladding + board cladding | 74.00% | |
Model 10: volume ~ working days + floor area + bathrooms + corners + type of builder + sheet cladding + board cladding | 78.00% |
Table 5.
Principal component analysis of built area for subset2.
Table 5.
Principal component analysis of built area for subset2.
PC1 | PC2 | |
---|---|---|
Eigenvalue | 3.57 | 0.30 |
Variance explained | 89.93 | 7.41 |
Loading scores: | ||
Floor area | −0.52 | −0.33 |
External perimeter | −0.51 | 0.29 |
Internal wall length | −0.48 | 0.67 |
Roof area | −0.49 | −0.60 |
Table 6.
Generalised linear model (model 2) without roof parameters.
Table 6.
Generalised linear model (model 2) without roof parameters.
Estimate | Std. Error | t Value | p Value | |
---|---|---|---|---|
(Intercept) | 0.609 | 0.165 | 3.682 | 0.000 |
Working days | 0.003 | 0.001 | 4.103 | 0.000 |
Floor area | 0.002 | 0.001 | 2.741 | 0.007 |
Internal wall length | −0.001 | 0.001 | −0.463 | 0.644 |
External perimeter | 0.002 | 0.003 | 0.755 | 0.451 |
Board cladding area | 0.000 | 0.001 | 0.334 | 0.739 |
Sheet cladding area | 0.000 | 0.001 | 0.710 | 0.479 |
Brick or stone cladding area | 0.001 | 0.001 | 1.313 | 0.191 |
Number of stories—2 | 0.094 | 0.092 | 1.017 | 0.311 |
Number of bathrooms | ||||
2 | −0.183 | 0.127 | −1.442 | 0.151 |
2.5 | −0.286 | 0.143 | −1.994 | 0.048 |
3 | −0.162 | 0.142 | −1.141 | 0.256 |
3.5 | −0.236 | 0.170 | −1.388 | 0.167 |
4 | −0.202 | 0.230 | −0.875 | 0.383 |
House type | 0.073 | 0.065 | 1.135 | 0.258 |
Number of corners | 0.008 | 0.004 | 2.212 | 0.029 |
Builder type—Small builders | 0.367 | 0.076 | 4.861 | 0.000 |
Table 7.
Best-fitting generalised linear model (model 3) for waste volume with roof parameters.
Table 7.
Best-fitting generalised linear model (model 3) for waste volume with roof parameters.
Estimate | Std. Error | t Value | p Value | |
---|---|---|---|---|
(Intercept) | 2.00 | 0.02 | 95.60 | 0.00 |
Stories—2 | 0.26 | 0.08 | 3.35 | 0.00 |
Sheet cladding area | 0.00 | 0.00 | 1.79 | 0.08 |
PC1 | −0.09 | 0.01 | −10.42 | 0.00 |
PC2 | −0.05 | 0.02 | −2.15 | 0.04 |
Table 8.
Generalised linear model (model 4) with roof parameters.
Table 8.
Generalised linear model (model 4) with roof parameters.
Estimate | Std. Error | t Value | p Value | |
---|---|---|---|---|
(Intercept) | 2.040 | 0.044 | 45.989 | 0.000 |
Working days | 0.000 | 0.000 | −0.237 | 0.814 |
PC1 | −0.095 | 0.017 | −5.460 | 0.000 |
PC2 | −0.060 | 0.039 | −1.520 | 0.138 |
Roof cladding type | −0.003 | 0.026 | −0.123 | 0.903 |
Sheet cladding area | 0.000 | 0.000 | 1.672 | 0.104 |
Number of bathrooms | ||||
2.5 | −0.044 | 0.025 | −1.800 | 0.081 |
3 | −0.043 | 0.157 | −0.274 | 0.786 |
3.5 | 0.050 | 0.098 | 0.509 | 0.614 |
Number of stories—2 | 0.217 | 0.154 | 1.415 | 0.166 |
Table 9.
Predicted values from ensemble models of the prediction tool.
Table 9.
Predicted values from ensemble models of the prediction tool.
Model 2 | Model 3 | Average Predictions | Actual Waste Volume | ||||||
---|---|---|---|---|---|---|---|---|---|
Prediction | Lower Limit | Upper Limit | Prediction | Lower Limit | Upper Limit | Prediction | Lower Limit | Upper Limit | |
6.96 | 5.75 | 8.42 | 7.10 | 6.44 | 7.83 | 7.03 | 6.10 | 8.13 | 7.00 |
6.81 | 5.82 | 7.96 | 7.68 | 7.14 | 8.26 | 7.24 | 6.48 | 8.11 | 7.25 |
6.76 | 5.81 | 7.88 | 7.38 | 6.91 | 7.89 | 7.07 | 6.36 | 7.88 | 7.00 |
7.17 | 6.16 | 8.35 | 7.55 | 7.02 | 8.11 | 7.36 | 6.59 | 8.23 | 7.25 |
6.08 | 5.23 | 7.06 | 6.67 | 6.06 | 7.35 | 6.38 | 5.65 | 7.21 | 6.50 |
6.88 | 5.69 | 8.32 | 7.06 | 6.42 | 7.76 | 6.97 | 6.06 | 8.04 | 7.50 |
8.11 | 6.93 | 9.49 | 8.09 | 7.47 | 8.76 | 8.10 | 7.20 | 9.13 | 8.00 |
7.68 | 6.48 | 9.10 | 6.94 | 6.36 | 7.57 | 7.31 | 6.42 | 8.33 | 7.50 |
Disclaimer/Publisher’s Note: The statements, opinions and data contained in all publications are solely those of the individual author(s) and contributor(s) and not of MDPI and/or the editor(s). MDPI and/or the editor(s) disclaim responsibility for any injury to people or property resulting from any ideas, methods, instructions or products referred to in the content. |
[ad_2]