Is Alexa Happy or Angry? Perceptions and Attributions of Emotional Displays of Smart Technologies in Residential Homes
[ad_1]
1. Introduction
In the remainder of this paper, we first introduce research on attribution theory and emotions in computers and virtual assistants and advance five hypotheses about users’ social cognitions, including two hypotheses about the role of distinctiveness in attributions that are informed by Kelley’s covariance theory. Next, we report two studies that tested the proposed hypotheses and identified conditions that triggered the described three basic social cognitions. Whereas Study 1 tested the first three hypotheses related to the proposed basic social cognitions, Study 2 included the hypotheses on distinctiveness. We conclude by discussing the limitations of the provided studies and offering questions for future research.
2. The Scenario of Residential Internet of Things (IoT): Attributional Outcomes
To study how actors and observers attribute emotional displays of virtual assistants, a context was defined in which individuals would utilize a virtual assistant in order to manipulate an IoT device. In this temperature regulation scenario, individuals imagine they are residents of an apartment complex and regulate their residential temperature by making requests for the virtual assistant to manipulate the temperature via a thermostat in the virtual assistant’s control. Individuals in a residence can ask for a variety of temperature changes and can make such requests multiple times as they decide to regulate the temperature of their residence. In response to the users’ requests, a virtual assistant can proffer a confirmation of a temperature change, provide extraneous information to a user’s request, and can display a facsimile of an emotional state.
3. The Display of Emotions by Virtual Assistants
To have the intended effect on residents’ behavior, emotional displays have to be identified by residents. Thus, producing effects of emotional displays on energy-saving behaviors of residents entails the task of designing a variety of distinguishable emotional displays. The virtual assistant has to display emotions in a way such that observers can correctly identify the portrayed emotion of the assistant. This task is not trivial, as non-verbal auditory communication can complement or contradict verbally stated emotions. As part of this project, we developed a method to program a virtual assistant in a way that would display the emotion of happiness and anger. The virtual assistant was an Amazon Alexa, programmed with different voices to appear as a unique virtual assistant. Two male voices and two female voices were used. Happiness and anger conditions were varied through the virtual assistant’s response to user requests, where the response contained an expression of happiness or anger through the use of semantics, rate of speech, volume, and pitch.
In happiness conditions, the virtual assistant said “I am happy with…” with an increase in pitch (out of three options in Amazon’s Speech Synthesis Markup Language), an increase in volume, and at 5% reduction in speed. In anger conditions, the virtual assistant said “I am annoyed with…” with a decrease in pitch (out of three options in Amazon’s Speech Synthesis Markup Language), an increase in volume, and a 5% reduction in speed.
As one important goal, the study aimed to find out if the emotional displays of happiness and anger of an Alexa that is programmed in this way and explicitly expresses that it is happy or angry about an object will be correctly identified, yielding the first hypothesis:
Emotional displays of happiness and anger that are programmed through the use of semantics, rate of speech, volume, and pitch are identified and recognized by users of Alexa: happy displays are being judged as happier than angry displays and vice versa.
4. Emotional Display Targets and Attributions
4.1. Hillebrandt and Barclay’s Study
This would imply that, when faced with emotional displays from a virtual assistant, individuals are more likely to attribute the display of emotion to a user’s behavior when the emotional display has an integral target. Specifically, in an interaction with a virtual assistant wherein a user makes a request of the virtual assistant, one such integral target would be the user’s request. In such a situation, Hillebrandt and Barclay’s findings suggest that individuals are more likely to attribute the virtual assistant’s emotional display to the user’s behavior—namely, the request the user made—than in a situation in which the emotional display has an incidental target.
This rationale yielded the following hypotheses:
Incidental and integral targets of an emotional display can be correctly identified and remembered by at least 90% of users of a virtual assistant and observers of an interaction of a user with a virtual assistant.
If a virtual assistant’s emotional display targets a specific request and is, thus, integral to an interaction, individuals will be more likely to attribute the cause of the emotional display to the specific request, in comparison to when the target of a virtual assistant’s emotional display appears incidental to the interaction.
4.2. Kelley’s Covariance Theory: The Role of Distinctiveness
Of the three dimensions proposed by Kelley, distinctiveness addresses both the temporal nature of information influencing attributions as well as the variability in potential causes which an emotional display can be attributed to. As such, a primary focus of the current work towards predicting how emotional displays are attributed over time was in the understanding of the role of distinctiveness and how distinctiveness interacts with the manipulated target.
If the virtual assistant’s emotional displays are distinct (with regard to the user or an integral or incidental target), observers of the assistant’s interactions with users recognize the dimension of distinctiveness (in terms of the user or an integral or incidental target) in the displayed emotions.
If a virtual assistant’s emotional display targets a specific request in a distinct way, individuals will be more likely to attribute the cause of the emotional display to the specific request, in comparison to when the target of a virtual assistant’s emotional display appears incidental to the interaction or when the emotional display is not distinct.
5. Overview of Current Research
To test the proposed hypotheses and to explore the role of dispositional attributions, we conducted two studies that included a variety of different measures of participants’ attributions of Alexa’s emotional displays. Both studies involved a scenario wherein users interacted with a virtual assistant to regulate the temperature of their residence. Participants were put in the role of an observer, watching videos of confederates interacting with a virtual assistant. The studies set out to test whether individuals are able to recognize the emotional display of Alexa and the target of Alexa’s messages. In addition, the studies aimed to find out when individuals make dispositional attributions and when individuals make situational attributions while observing virtual assistants’ emotional displays to test for a relationship between the target of a virtual assistant’s emotional displays and attributions to user requests.
Even though the studies focused on situational attributions, we also included measures on dispositional attributions. Situational attributions attribute a behavior, including the display of an emotion, to causes that are rooted in the situation in which the behavior is shown. Attributing an emotion to a specific request would be an example of a situational attribution. Conversely, dispositional attributions attribute a behavior to the disposition of the actor or the observer.
6. Study 1
6.1. Methods
6.1.1. Participants and Design
For Study 1, the initial sample was 232 participants. Seventeen participants were not included in the analysis due to failing the attention check, and 21 participants were removed for failing to complete the study, leaving a sample of 194 participants. Of these participants, 126 were male, 66 were female, and 2 chose not to answer. The age of participants ranged from 23 to 78 years with M = 36.74 (SD = 10.92). In terms of education, 7.2% of the participants had graduated from high school or had a GED; 8.8% indicated that they had some college education; 36.6% had a bachelor’s degree; 5.7% had attended some graduate school; 40.7% had a graduate degree; and 1% chose not to answer.
The study varied two factors. First, the emotional display of the virtual assistant was either angry or happy. Second, the emotional display either targeted a request by the user or extraneous information (integral vs. incidental target). The extraneous information referred to HBO or something on the news. The gender emulated by the voice of the virtual assistant included male as well as female speakers. Factors were manipulated in a between-subjects design. As dependent variables, the study measured perceived emotion and target and included a forced-choice measure of primary attribution and an instrument measuring attributions to specific requests.
6.1.2. Procedure
6.1.3. Materials
The study was administered through Qualtrics. The videos showed confederates interacting with a virtual assistant. The virtual assistant was an Amazon Alexa, programmed with different voices to appear as a unique virtual assistant. Two male voices and two female voices were used. Happiness and anger conditions were varied through the virtual assistant’s response to user requests, where the response contained an expression of happiness or anger through the use of semantics, rate of speech, volume, and pitch. Target was varied through the virtual assistant indicating that it was either happy or angry about the temperature change (integral target) or the extraneous information provided by the virtual assistant (incidental target). Two pieces of extraneous information were used to separate the impact of extraneous information from the impact of incidental targets (the virtual assistant telling the confederate that “By the way, HBO got new movies” or “By the way, I got a breaking news update”).
6.1.4. Measures
An additional forced-choice item asked participants to identify which of the following most likely caused the virtual assistant’s display of emotion: (a) a specific request made by the user, (b) the user regardless of request, (c) the virtual assistant’s programming, (d) HBO’s new movies, or (e) the news.
6.2. Results
6.2.1. Emotion
Hypothesis 1 predicted that participants judge the emotional display in the happy condition to be happier than in the angry condition; likewise, the emotional display in the angry condition was expected to be judged to appear angrier than the display in the happy condition. As perceived happiness and anger were measured independently, two ANOVAs were conducted comparing the respective scores for happiness and anger between conditions for emotion and target. Results showed that the variation of the virtual assistant’s display of emotion was effective. Participants in the happy condition reported higher perceived happiness (M = 3.82, SD = 0.84) than those in the anger condition (M = 3.28, SD = 1.38) in the analysis of perceived happiness (main effect emotion, F(1,190) = 9.88, p = 0.002, ηp2 = 0.05). Likewise, participants in the happy condition reported lower perceived anger (M = 2.52, SD = 1.31) than those in the anger condition (M = 3.62, SD = 1.12) in the analysis of perceived anger (main effect emotion, F(1,190) = 38.45, p < 0.001, ηp2 = 0.17). The target manipulation did not significantly impact perceptions of happiness (all Fs < 1.18) or anger (all Fs < 0.49).
6.2.2. Targets
While 73 (82.02%) participants in the incidental target condition correctly identified incidental targets, only 44 (41.90%) participants correctly identified integral targets. These findings were independent of the emotional display. Participants in the integral target happy condition made inferences about the target that were similar to those in the integral target angry condition. In the integral target happy condition, correct identification was achieved by 21 (42%) participants, and in the incidental target happy condition, 34 (80.95%) participants correctly identified the emotional display target, while 23 (41.82%) participants in the integral target angry condition and 39 (82.98%) participants in the incidental target angry condition correctly identified the target. Neither the used gender of Alexa nor the variation of extraneous information had an effect on perceived emotion or the perception of targets. Thus, Hypothesis 2 was not supported. Many participants had difficulty identifying and remembering when Alexa referred to the specific temperature change request.
6.2.3. Attributions
Overall, 65 (33.51%) participants chose a specific request as the primary attribution, 19 (9.79%) chose the user, 71 (36.60%) chose the virtual assistant, and 39 (20.10%) chose the extraneous information the virtual assistant provided. When split by emotion, participants were more likely to attribute the cause of the virtual assistant’s emotions to the virtual assistant when it was angry (44, 43.10%) and more likely to attribute it to a specific request when it was happy (27, 29.30%) (anger: χ2(3, 102) = 23.02, p < 0.001; happiness: χ2(3, 92) = 20.52, p < 0.001).
Hypothesis 3 suggested that participants in the integral target condition would make greater attributions to a specific request than those in the incidental target condition. When the virtual assistant responded to user requests with an emotional display that targeted their request (the integral target condition), participants’ attributions to the specific request averaged M = 3.57 (SD = 1.04) across the three items used to assess attributions to specific requests. When the virtual assistant targeted information extraneous to the user’s request (the incidental target condition), participants’ attributions to the specific request averaged M = 3.34 (SD = 1.26). The predicted pattern held for the happy condition wherein the means for those in integral vs. incidental target conditions were significantly different (integral: M = 3.77, SD = 0.81; incidental: M = 3.38, SD = 1.19; t(67.97) = 2.18, p = 0.02) but not for the angry condition (integral: M = 3.38, SD = 1.19; incidental: M = 3.39, SD = 1.29; t(100) = 0.06, p = 48). A two-way ANOVA comparing the effect of target and emotion on attributions to a specific request resulted in insignificant main effects for target (F(1,190) = 2.06, p = 0.15) and emotion (F(1,190) = 0.791, p = 0.38), with no significant interaction between the two (F(1,190) = 2.32, p = 0.13). As participants in the happy condition had made significantly greater attributions to specific requests to emotional displays with integral targets compared to those which had incidental targets, Hypothesis 3 was partially supported.
An additional test of Hypothesis 3 was conducted through assessing the impact of target and emotion on the forced-choice single item question, asking participants to choose the most likely cause of the virtual assistant’s emotional display. When attributions to the two extraneous information sources were condensed into one category, 38 (36.19%) participants in the integral target condition selected user requests as the primary cause of the emotional display, and 27 (30.34%) participants in the incidental target condition selected user request as the primary cause attributed to the emotional display.
As participants had low accuracy in identifying targets, we also tested Hypothesis 3 on participants who were able to correctly identify the target of the virtual assistant’s emotional display (N = 117). Within this group of participants, the average score for attributions to the specific request in the integral target condition (M = 3.31; SD = 1.02) did not systematically differ from the score in the incidental target condition (M = 3.25; SD = 1.32; t(170.58) = 0.29, p = 0.39), resembling the findings for the whole study sample.
6.3. Discussion
Study 1 demonstrated how a virtual assistant’s emotional displays of happiness and anger can be manipulated such that they are identified and recognized by individuals who watch an interaction of an Alexa user with the virtual assistant. This is an important requirement to be able to influence energy-saving behaviors of residents through emotional displays. At the same time, the study revealed that only about 40% of observers correctly identified a user’s request to change the room temperature as the target of an emotional display when Alexa explicitly referred to it while expressing an emotion (integral target condition). As suggested by the literature on attributions concerning human-computer interactions, a significant percentage of attributions were dispositional (36%). Together, attributions towards the virtual assistant and the specific user request accounted for 70% of the chosen attributions. Hypothesis 3 predicted that emotional displays that are integral to an interaction will be more likely attributed to the specific request of the conversation than displays that have an incidental target. There was some support for the hypothesis for happy displays but not for angry displays.
7. Study 2
Study 2 had the goal to test all five proposed hypotheses, including the hypotheses on distinctiveness. Study 2 extended Study 1 by having participants watch not only one video, but a series of videos of three users interacting with the virtual assistant prior to watching a final video of a user–assistant interaction. Participants were then asked to make attributions of cause to the emotional display from the virtual assistant in the final video. This was done in order to assess whether changes in the distinctiveness with which situational features co-occur with the virtual assistant’s emotional displays over subsequent interactions impact participants’ attributions. The use of multiple videos allowed participants to observe combinations of situational features, with emotional displays only occurring when a specific situational feature was present. Specifically, the sequence of videos made it so that either a specific user request, a specific user, or a specific piece of extraneous information (an HBO, news, or software update) was the most distinct situational feature co-occurring with videos containing an emotional display from the virtual assistant. As gender had not significantly impacted attributions in Study 1, it was dropped as a variable for the present study. Study 2 also served the purpose of replicating the main findings of Study 1.
7.1. Methods
7.1.1. Participants and Design
For Study 2, the initial sample had 458 participants. The final sample had 353 participants, as 54 were not included in the analysis due to failing the attention check, and 51 were removed for failing to complete the study. There were 227 male participants, 124 female participants, and 2 chose not to indicate their gender. The age of participants ranged from 20 to 76 years (M = 36.17, SD = 10.12). In terms of education, 6.2% of the participants had graduated from high school or had a GED; 8.5% indicated that they had some college education; 35.4% had a bachelor’s degree; 7.1% had attended some graduate school; 42.5% had a graduate degree; and 0.3% chose not to answer. As with Study 1, participants were compensated USD 1.82 for taking part in the approximately 15 min-long study.
The design had three factors: emotion (happiness vs. anger), target (integral vs. incidental), and distinctiveness (distinct user vs. distinct request vs. distinct extraneous information vs. indistinct). In addition, the study used three different forms of extraneous information (HBO vs. news vs. software). The same measures of attribution (scales for each potential attribution plus a forced choice measure) were used, as in Study 1.
7.1.2. Procedure
As with Study 1, participants were recruited through Amazon Mechanical Turk to take part in a study administered through Qualtrics that tested their perceptions of a virtual assistant. Participants were given the same task introduction as in Study 1 but were told that they would be watching a series of interactions between residents and a virtual assistant before being asked questions about a final interaction. Participants then watched a series of 19 videos where users made requests of the virtual assistant. The number of videos was a result of three users each making six requests of the virtual assistant, followed by a final video which participants were asked questions about.
Four levels of distinctiveness were designed (distinct user, distinct request, distinct extraneous information, and indistinctiveness) to account for variance in potential situational causes over the course of multiple interactions between the virtual assistant and the three users. For each distinctiveness condition, a different situational feature was always accompanied by an emotional display which only occurred when that situational feature was present. The exception was the indistinctiveness condition, in which the virtual assistant used an emotional display in every video.
The use of three users allowed for a condition wherein only one user was responded to with emotional displays. Each user made three requests twice, with each repeated request accompanied by a differing piece of extraneous information, which allowed one of the three requests to be the most distinctive feature in the distinct request condition. The use of three pieces of extraneous information (as opposed to two pieces from Study 1) allowed each piece of extraneous information to be accompanied by two different requests, and thus be the most distinctive situational feature in distinct extraneous information conditions. Using the distinct request condition as an example, across the first 18 videos, participants observed the virtual assistant using emotional displays in six videos, twice for each user and with two differing pieces of extraneous information for each user.
Each video featured a user asking the virtual assistant for one of three temperature changes and receiving a response from the virtual assistant which, as with Study 1, included an acknowledgement of the request, an emotional display, and a piece of extraneous information. As an example of the integral target distinct extraneous information condition, a single video might contain a request to change the temperature to 72°, an acknowledgement of the temperature change, the virtual assistant telling the user it received an HBO update, and then the virtual assistant indicating that it was happy with the new movies. For each distinctiveness condition, if the situational feature was not present, then the video did not contain an emotional display. The order of the videos was randomized across participants. Participants were then given one last video to answer questions about, which contained the situational feature which had distinctly covaried with the emotional display during the 18 videos.
Following that final nineteenth video, participants were asked a series of questions about their perceptions of emotion in the virtual assistant’s response including the perceived emotion and target of the display and its distinctiveness and possible attributions.
7.1.3. Materials and Measures
Study 2 used the same materials as Study 1, albeit with the inclusion of more videos as per the procedure. The same measures for perceived emotion and perceived target were used in Study 2 as were used in Study 1 (happiness scale: Cronbach’s α = 0.88; anger scale: Cronbach’s α = 0.89).
7.2. Results
7.2.1. Emotion
As with Study 1, two ANOVAs were conducted comparing the respective scores for anger and happiness between conditions for emotion, target, and distinctiveness for Study 2. The variation of the virtual assistants’ emotional displays was effective, and Hypothesis 1 was supported. Participants in the happy condition reported higher perceived happiness (M = 3.77, SD = 0.89) than those in the anger condition (M = 3.27, SD = 1.14), and the analysis checking participants’ perceptions of happiness yielded a main effect for emotion displayed, F(1,337) = 25.26, p < 0.001, ηp2 = 0.07. Likewise, participants in the happy condition reported lower perceived anger (M = 2.70, SD = 1.27) than did those in the anger condition (M = 3.57, SD = 0.92), and the analysis using perceived anger as a dependent variable yielded a main effect for emotion F(1,337) = 43.89, p < 0.001, ηp2 = 0.12. Thus, Hypothesis 1 was supported.
7.2.2. Target
An analysis of participants’ identification of targets revealed that integral targets were correctly identified in 115 cases (64.61%), and incidental targets were correctly identified in 102 cases (58.29%). This held for both happiness and anger conditions, as 57 (66.28%) participants in the integral target happy condition and 51 (58.62%) participants in the incidental target happy condition correctly identified the target, while 58 (63.04%) participants in the integral target angry condition and 51 (57.95%) participants in the incidental target angry condition correctly identified the target. Thus, different from Study 1, the identification of integral and incidental targets did not systematically differ from each other. As more than one-third of participants did not correctly remember the target of the emotional displays, the 90% criterion of Hypothesis 2 was not met.
7.2.3. Distinctiveness
Participants’ perceptions of distinctiveness were measured on four levels: the degree to which the virtual assistant responded differently to distinct requests, distinct users, distinct extraneous information, or indistinctly responded to all situational features. Except for the distinct request condition, the means for participants’ perceptions of distinctiveness were highest for the feature that was most distinct (distinct request, distinct extraneous information, or distinct user), and perceptions of no distinct specific request, specific user, or extraneous information (indistinctiveness) were highest in the indistinctiveness condition.
In the distinct request condition, participants did not perceive a greater degree of distinctiveness in Alexa’s responses to users’ specific requests (M = 3.33, SD = 1.09) than those in other conditions (M = 3.36, SD = 1.04) F(3,337) = 0.34, p = 0.79. In the distinct user condition, participants perceived a greater degree of distinctiveness of Alexa’s responses between users (M = 3.70, SD = 1.13) than those in other distinctiveness conditions (M = 3.32, SD = 1.23) F(3,337) = 3.62, p = 0.01, ηp2 = 0.03. In the distinct extraneous information condition, participants reported a greater degree of distinctiveness in Alexa’s responses to different types of extraneous information (M = 3.76, SD = 0.99) than in the other distinctiveness conditions (M = 3.22, SD = 1.15) F(3,337) = 4.92, p = 0.002, ηp2 = 0.04. Finally, in the indistinct condition, participants perceived a greater degree of indistinctiveness in Alexa’s responses (M = 3.58, SD = 1.16) than they did in other distinctiveness conditions (M = 3.11, SD = 1.29) F(3,337) = 4.33, p = 0.01, ηp2 = 0.04. Thus, Hypothesis 4 was partially supported. Participants were sensitive to distinctiveness in the responses of Alexa and perceived greater distinctiveness for the variation across users and extraneous information. However, Alexa’s responses to specific requests by users were not perceived as being more distinct.
7.2.4. Attributions
In Study 2, 97 (27.48%) participants chose a specific request as the primary attribution; 32 (9.07%) chose the user; 138 (39.09%) chose the virtual assistant; and 86 (24.36%) chose the extraneous information the virtual assistant provided. Participants averaged M = 3.36 (SD = 1.25) in their attributions to specific requests; M = 3.22 (SD = 1.12) in their attributions to extraneous information; M = 3.63 (SD = 1.02) in their attributions to Alexa’s disposition; and M = 3.24 (SD = 1.25) in their attributions to a specific user.
7.3. Discussion
Study 2 replicated the test of Hypothesis 1 from Study 1 indicating that participants were able to distinguish between happy and angry emotional displays. Even though more participants correctly identified the intended integral target in Study 2 than in Study 1, Hypothesis 2 was again not supported as more than one-third of participants selected a wrong target.
Emotion target had a main effect on attributions to requests. Distinctiveness was found to significantly impact attributions to specific users in the angry condition but did not significantly impact attributions to specific requests or the virtual assistant. These findings, as well as limitations of the study which qualify these findings, are discussed below.
Whereas Study 1 had only found an effect for emotion targets on attributions in the happy condition, emotion targets had significant impacts on attributions to specific requests regardless of emotion over repeated interactions and had a marginally significant impact on attributions to the virtual assistant’s disposition in Study 2. This suggests that repeated experience may amplify the effect of emotion targets. It also suggests that the impact of integral emotion targets on user attributions can matter even in the face of situational evidence. Distinctiveness had a significant effect on the degree to which individuals attributed angry displays of emotion to specific users. A post-hoc test comparing all combinations of emotion target and distinctiveness showed a significant difference in attribution requests between integral and incidental targets for the distinct request condition.
Distinctiveness appears to affect situational attributions to virtual assistant’s emotional displays based on the emotion being displayed but has limited effects on dispositional attributions. This does provide support for the assumption that it is not just Kelley’s covariances, but also the content of communication, that influences attributions.
8. Conclusions
The reported studies have implications for our understanding of the role of emotional targets, Kelley’s attribution theory, and human–computer interactions with virtual assistants such as Alexa. The studies suggest that emotional display targets can affect observer attributions and that repeated exposure can make the attributions suggested by these targets more convincing to observers.
On the whole, participants were able to identify the presented emotional displays, which provides an important step for future research. Moreover, Study 1 and Study 2 both found significant differences between integral and incidental targets for happiness. Additionally, anger qualified the distinctiveness perception in Study 2 and anger displays were more recognizable to participants than happy displays, based on perceived emotions across both studies. As such, there may also be expectations about virtual assistant’s use of emotions that have built norms over how happiness is displayed and directed by virtual assistants. In future studies, it would be interesting to investigate the role of expectations in attributions of emotional displays, such as whether the attributional impact of unusual and unexpected emotional behaviors from virtual assistants differs from expected displays.
As a prerequisite, to have an intended effect on energy saving behaviors, Alexa’s emotional messages have to trigger at least three basic social cognitions: (1) the emotional displays (e.g., the expression of happiness or anger) have to be identified by residents; (2) residents have to correctly identify user inputs as a target of the emotional display; and (3) residents have to attribute the emotional display to that behavior. The described studies identified conditions that triggered these three basic social cognitions in a simulated environment. Whereas the variation and implementation of the emotional displays of happiness and anger were successful across various conditions in both studies (1), more research is needed to identify more effective references to integral targets that are perceived and remembered by potential residents (2), and to identify and delineate more effective and robust methods to trigger inferences that attribute Alexa’s emotional displays to a specific request if this is the focal target of Alexa’s emotional display (3).
Author Contributions
Conceptualization, H.B., T.R. and J.R.; Methodology, H.B. and T.R.; Software, H.B. and D.Z.; Validation, H.B. and D.Z.; Formal analysis, H.B. and T.R.; Resources, H.B. and D.Z.; Writing—original draft, H.B. and T.R.; Writing—review & editing, H.B., T.R and J.R.; Funding acquisition, T.R. and J.R. All authors have read and agreed to the published version of the manuscript.
Funding
This work was supported by the National Science Foundation under Grant NSF1737591 to Torsten Reimer and Julia Rayz.
Institutional Review Board Statement
The study was conducted in accordance with the Declaration of Helsinki and approved by the Institutional Review Board of Purdue University (protocol code IRB-2022-80).
Informed Consent Statement
Informed consent was obtained from all participants involved in the study.
Data Availability Statement
The data presented in this study are available on request from the corresponding author.
Conflicts of Interest
The authors declare no conflict of interest.
References
- Hoy, M.B. Alexa, Siri, Cortana, and more: An introduction to voice assistants. Med. Ref. Serv. Q. 2018, 37, 81–88. [Google Scholar] [CrossRef]
- Johnson, N.; Reimer, T. The adoption and use of smart assistants in residential homes: The matching hypothesis. Sustainability 2023, 15, 9224. [Google Scholar] [CrossRef]
- Kim, H.; Ham, S.; Promann, M.; Devarapalli, H.; Bihani, G.; Ringenberg, T.; Kwarteng, V.; Bilionis, I.; Braun, J.E.; Taylor Rayz, J.; et al. MySmartE—An eco-feedback and gaming platform to promote energy conserving thermostat-adjustment behaviors in multi-unit residential buildings. Build. Environ. 2022, 221, 109252. [Google Scholar] [CrossRef]
- Ram, A.; Prasad, R.; Khatri, C.; Venkatesh, A.; Gabriel, R.; Liu, Q.; Nunn, J.; Hedayatnia, B.; Cheng, M.; Nagar, A.; et al. Conversational ai: The science behind the Alexa prize. arXiv 2018, arXiv:1801.03604. [Google Scholar] [CrossRef]
- Edwards, A.; Edwards, C. Does the correspondence bias apply to social robots?: Dispositional and situational attributions of human versus robot behavior. Front. Robot. AI 2021, 8, 788242. [Google Scholar] [CrossRef]
- Van Kleef, G.A. The emerging view of emotion as social information. Soc. Personal. Psychol. Compass 2010, 4, 331–343. [Google Scholar] [CrossRef]
- Nass, C.; Steuer, J.; Tauber, E.R. Computers are social actors. In Proceedings of the SIGCHI Conference on Human Factors in Computing Systems, Boston, MA, USA, 24–28 April 1994; Volume 1, pp. 72–78. [Google Scholar] [CrossRef]
- Nass, C.; Steuer, J.; Tauber, E.; Reeder, H. Anthropomorphism, agency, and ethopoeia. In INTERACT’93 and CHI’93 Conference Companion on Human Factors in Computing Systems; ACM: New York, NY, USA, 1993; Volume 1, pp. 111–112. [Google Scholar] [CrossRef]
- Nass, C.; Moon, Y.; Fogg, B.J.; Reeves, B.; Dryer, D.C. Can computer personalities be human personalities? Int. J. Hum.-Comput. Stud. 1995, 43, 223–239. [Google Scholar] [CrossRef]
- Zhao, S. Humanoid social robots as a medium of communication. New Media Soc. 2006, 8, 401–419. [Google Scholar] [CrossRef]
- Loaiza-Ramírez, J.P.; Reimer, T.; Moreno-Mantilla, C.E. Who prefers renewable energy? A moderated mediation model including perceived comfort and consumers’ protected values in green energy adoption and willingness to pay a premium. Energy Res. Soc. Sci. 2022, 91, 102753. [Google Scholar] [CrossRef]
- Loaiza-Ramirez, J.P.; Moreno-Mantilla, C.E.; Reimer, T. Do consumers care about companies’ green supply chain management (GSCM) efforts? Analyzing the role of protected values and the halo effect in product evaluation. Clean. Logist. Supply Chain. 2022, 3, 100027. [Google Scholar] [CrossRef]
- Fogg, B.J.; Nass, C. Silicon sycophants: The effects of computers that flatter. Int. J. Hum.-Comput. Stud. 1997, 46, 551–561. [Google Scholar] [CrossRef]
- Ammari, T.; Kaye, J.; Tsai, J.Y.; Bentley, F. Music, search, and IoT: How people (really) use voice assistants. ACM Trans. Comput.-Hum. Interact. 2019, 26, 17. [Google Scholar] [CrossRef]
- Van Kleef, G.A. The Interpersonal Dynamics of Emotion; Cambridge University Press: Cambridge, UK, 2016. [Google Scholar]
- Hillebrandt, A.; Barclay, L.J. Comparing integral and incidental emotions: Testing insights from emotions as social information theory and attribution theory. J. Appl. Psychol. 2017, 102, 732–752. [Google Scholar] [CrossRef]
- Kelley, H.H. The processes of causal attribution. Am. Psychol. 1973, 28, 107–128. [Google Scholar] [CrossRef]
- Kelley, H.H.; Michella, J.L. Attribution theory and research. Annu. Rev. Psychol. 1980, 31, 457–501. [Google Scholar] [CrossRef]
- Martinko, M.J.; Thomson, N.F. A synthesis and extension of the Weiner and Kelley attribution models. Basic Appl. Soc. Psychol. 1998, 20, 271–284. [Google Scholar] [CrossRef]
- Shaver, K.G. An Introduction to Attribution Processes; Routledge: London, UK, 1983. [Google Scholar]
- Lichtenstein, D.R.; Bearden, W.O. Measurement and structure of Kelley’s covariance theory. J. Consum. Res. 1986, 13, 290–296. [Google Scholar] [CrossRef]
- Solomon, S. Measuring dispositional and situational attributions. Personal. Soc. Psychol. Bull. 1978, 4, 589–594. [Google Scholar] [CrossRef]
- Hovy, D.; Yang, D. The Importance of Modeling Social Factors of Language: Theory and Practice. In Proceedings of the 2021 Conference of the North American Chapter of the Association for Computational Linguistics: Human Language Technologies, Online, 6–11 June 2021; pp. 588–602. [Google Scholar] [CrossRef]
- Grice, H.P. Logic and conversation. In Speech Acts; Brill: Leiden, The Netherlands, 1975; pp. 41–58. [Google Scholar]
- Hortensius, R.; Hekele, F.; Cross, E.S. The Perception of Emotion in Artificial Agents. IEEE Trans. Cogn. Dev. Syst. 2018, 10, 852–864. [Google Scholar] [CrossRef]
- Davis, S.K.; Morningstar, M.; Dirks, M.A.; Qualter, P. Ability emotional intelligence: What about recognition of emotion in voices? Personal. Individ. Differ. 2020, 160, 109938. [Google Scholar] [CrossRef]
- Lei, X.; Rau, P.L.P. Should I blame the human or the robot? Attribution within a human–robot group. Int. J. Soc. Robot. 2021, 13, 363–377. [Google Scholar] [CrossRef]
- Li, Z.; Rau, P.L.P. Effects of self-disclosure on attributions in human–IoT conversational agent interaction. Interact. Comput. 2019, 31, 13–26. [Google Scholar] [CrossRef]
- Li, Z.; Rau, P.L.P. Talking with an IoT-CA: Effects of the use of internet of things conversational agents on face-to-face conversations. Interact. Comput. 2021, 33, 238–249. [Google Scholar] [CrossRef]
- Serenko, A. Are interface agents scapegoats? Attributions of responsibility in human–agent interaction. Interact. Comput. 2007, 19, 293–303. [Google Scholar] [CrossRef]
- Lee, K.M.; Nass, C. Social-psychological origins of feelings of presence: Creating social presence with machine-generated voices. Media Psychol. 2005, 7, 31–45. [Google Scholar] [CrossRef]
- Watkins, H.; Pak, R. Investigating user perceptions and stereotypic responses to gender and age of voice assistants. In Proceedings of the Human Factors and Ergonomics Society Annual Meeting; Sage: Los Angeles, CA, USA, 2020; Volume 64, pp. 1800–1804. [Google Scholar]
- Breazeal, C.; Aryananda, L. Recognition of affective communicative intent in robot directed speech. Auton. Robot. 2002, 12, 83–104. [Google Scholar] [CrossRef]
- Edwards, C.; Edwards, A.; Spence, P.R.; Westerman, D. Initial interaction expectations with robots: Testing the human-to-human interaction script. Commun. Stud. 2016, 67, 227–238. [Google Scholar] [CrossRef]
- Silberman, M.S.; Tomlinson, B.; LaPlante, R.; Ross, J.; Irani, L.; Zaldivar, A. Responsible research with crowds: Pay crowdworkers at least minimum wage. Commun. ACM 2018, 61, 39–41. [Google Scholar] [CrossRef]
- Tamborini, R.; Grall, C.; Prabhu, S.; Hofer, M.; Novotny, E.; Hahn, L.; Klebig, B.; Kryston, K.; Baldwin, J.; Aley, M.; et al. Using attribution theory to explain the affective dispositions of tireless moral monitors toward narrative characters. J. Commun. 2018, 68, 842–871. [Google Scholar] [CrossRef]
- Becker, C.; Kopp, S.; Wachsmuth, I. Why emotions should be integrated into conversational agents. In Conversational Informatics: An Engineering Approach; Nishida, T., Ed.; Wiley: Hoboken, NJ, USA, 2007; pp. 49–68. [Google Scholar]
- Toader, D.C.; Boca, G.; Toader, R.; Măcelaru, M.; Toader, C.; Ighian, D.; Rădulescu, A.T. The effect of social presence and chatbot errors on trust. Sustainability 2019, 12, 256. [Google Scholar] [CrossRef]
- Ahn, I.; Kim, S.H. Measuring the motivation: A scale for positive consequences in pro-environmental behavior. Sustainability 2023, 16, 250. [Google Scholar] [CrossRef]
- Mastria, S.; Vezzil, A.; De Cesarei, A. Going green: A review on the role of motivation in sustainable behavior. Sustainability 2023, 15, 15429. [Google Scholar] [CrossRef]
Figure 1.
Conceptual figure showing the proposed influence of message features from an Amazon Alexa. Note. The introduced hypotheses test assumptions of a framework that assumes that future user behaviors are not only affected by the content of feedback but also by the emotional display with which feedback is provided. Accordingly, emotional displays do not directly affect behavior but the exerted impact on behavior depends on how the observed emotional displays are attributed. Attributions are affected by the emotional displays as well as the targets of the displayed emotions and their distinctiveness.
Figure 1.
Conceptual figure showing the proposed influence of message features from an Amazon Alexa. Note. The introduced hypotheses test assumptions of a framework that assumes that future user behaviors are not only affected by the content of feedback but also by the emotional display with which feedback is provided. Accordingly, emotional displays do not directly affect behavior but the exerted impact on behavior depends on how the observed emotional displays are attributed. Attributions are affected by the emotional displays as well as the targets of the displayed emotions and their distinctiveness.
Figure 2.
Diagram illustrating how interaction and message features are expected to influence attributions of cause for emotional displays from an Amazon Alexa. Note: Study 1 manipulated emotional display targets in a video of a single interaction with a confederate, whereas Study 2 also manipulated distinctiveness of the emotional displays over the course of 19 videos. Conditions that were expected to facilitate attributions to user requests are highlighted in italics (see Hypotheses 3 and 5).
Figure 2.
Diagram illustrating how interaction and message features are expected to influence attributions of cause for emotional displays from an Amazon Alexa. Note: Study 1 manipulated emotional display targets in a video of a single interaction with a confederate, whereas Study 2 also manipulated distinctiveness of the emotional displays over the course of 19 videos. Conditions that were expected to facilitate attributions to user requests are highlighted in italics (see Hypotheses 3 and 5).
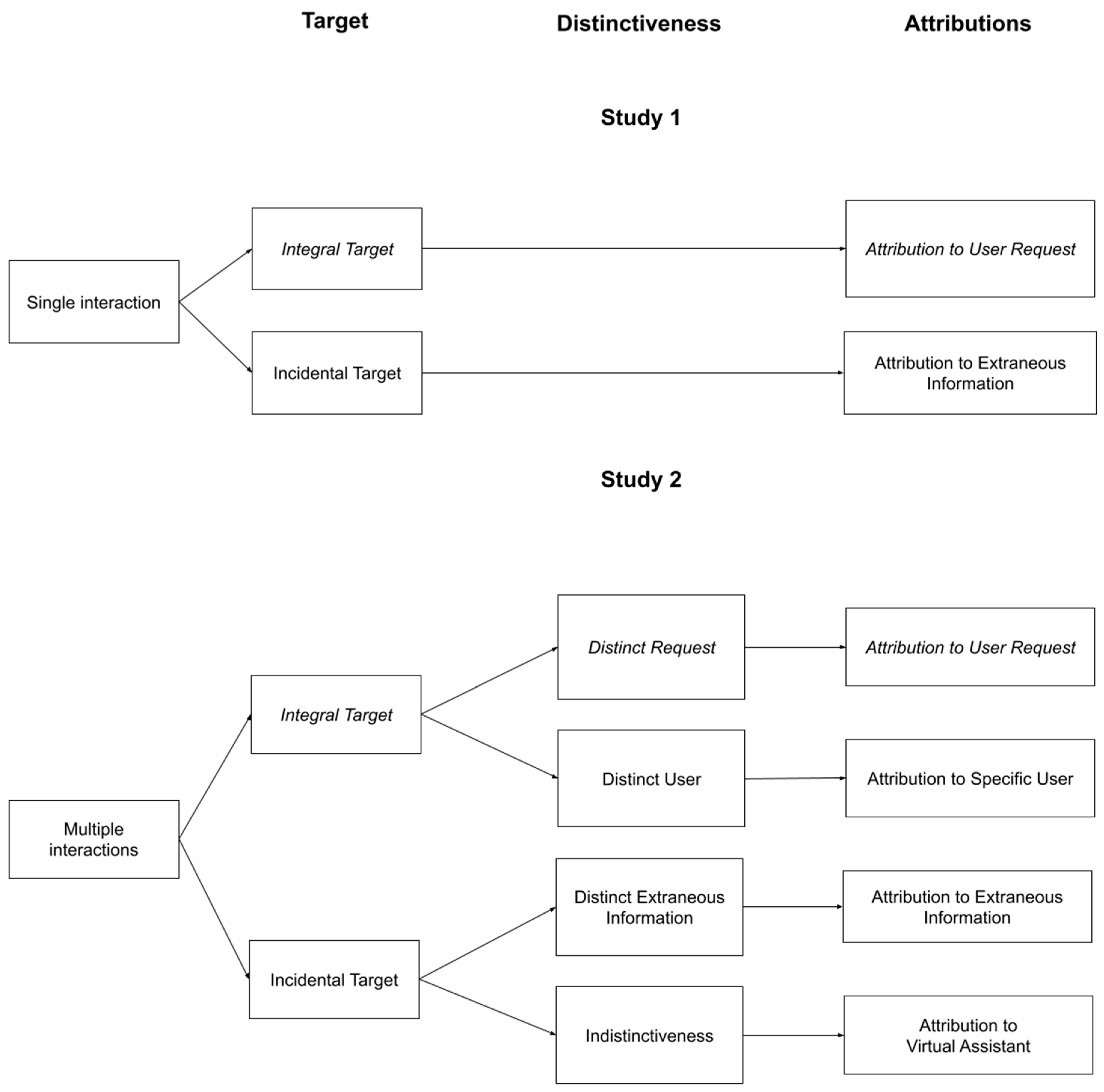
Table 1.
Study 2 attribution to specific request by condition.
Table 1.
Study 2 attribution to specific request by condition.
Design Conditions | Target | |
---|---|---|
Integral | Incidental | |
M (SD) | M (SD) | |
Distinct Request | ||
Happiness | 3.76 (0.73) | 2.94 (1.50) |
Anger | 3.97 (0.97) | 2.37 (1.42) |
Distinct Extraneous Information | ||
Happiness | 3.48 (1.14) | 3.54 (1.27) |
Anger | 3.72 (0.96) | 3.05 (1.53) |
Distinct user | ||
Happiness | 3.42 (1.29) | 2.71 (1.28) |
Anger | 3.65 (1.05) | 3.19 (1.22) |
Indistinct | ||
Happiness | 3.64 (1.07) | 2.69 (1.31) |
Anger | 3.73 (1.07) | 3.76 (1.17) |
Disclaimer/Publisher’s Note: The statements, opinions and data contained in all publications are solely those of the individual author(s) and contributor(s) and not of MDPI and/or the editor(s). MDPI and/or the editor(s) disclaim responsibility for any injury to people or property resulting from any ideas, methods, instructions or products referred to in the content. |
[ad_2]